Exploring the Vast Scope of Machine Learning
Discover machine learning, algorithms, data, AI, neural networks, deep learning, applications, technology, trends, tools, innovation
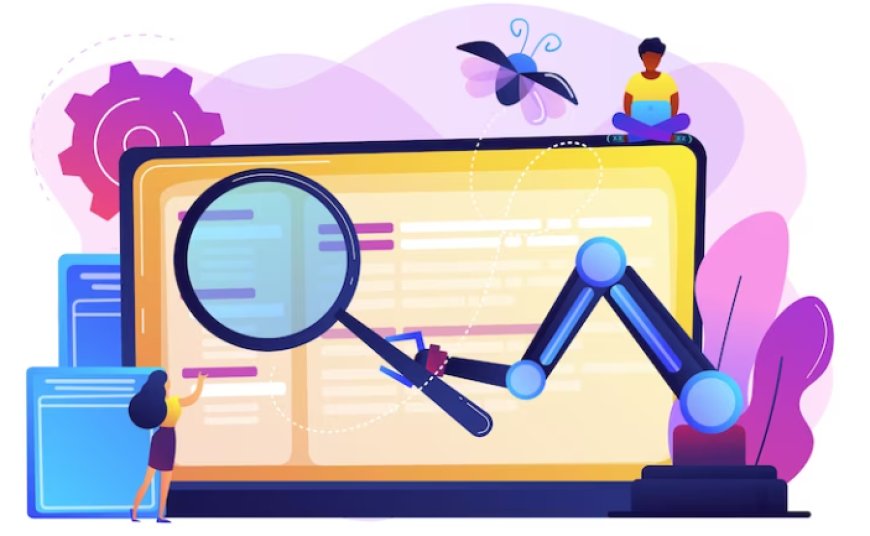
Machine learning has emerged as a transformative technology, revolutionizing various industries and reshaping the way we approach data-driven problem-solving. With its ability to learn patterns from data and make predictions or decisions, machine learning has a broad scope of applications across domains ranging from healthcare and finance to marketing and cybersecurity. In this blog, we will dive into the expansive scope of machine learning and explore the exciting possibilities it offers.
1. Healthcare:
Machine learning has become a game-changer in the field of healthcare, offering transformative opportunities to improve patient care, optimize treatment plans, and enhance medical research. By analyzing vast amounts of patient data, medical images, and genetic information, machine learning algorithms can assist in early disease detection, personalized treatment recommendations, and proactive disease prevention. Machine learning also plays a crucial role in real-time patient monitoring, anomaly detection, and predicting potential health risks. With its ability to extract valuable insights from healthcare data, machine learning is revolutionizing the industry and paving the way for more efficient, precise, and patient-centric healthcare delivery.
2. Finance:
Machine learning is reshaping the finance industry, bringing about significant advancements in decision-making, risk management, and financial analysis. By analyzing vast amounts of financial data, market trends, and customer behavior, machine learning algorithms can provide valuable insights for investment strategies, risk assessment, and fraud detection. Machine learning models are employed in credit scoring, algorithmic trading, and portfolio optimization, helping organizations make data-driven decisions and achieve better outcomes. With its ability to uncover hidden patterns and predict market trends, machine learning is revolutionizing finance, enabling more accurate predictions, improved risk management, and enhanced operational efficiency.
3. Marketing and Customer Analytics:
Machine learning has revolutionized the field of marketing and customer analytics by enabling businesses to extract valuable insights from vast amounts of data and make data-driven decisions. This technology leverages algorithms and statistical models to automatically learn patterns and make predictions, ultimately improving marketing strategies and enhancing customer experiences.
In marketing, machine learning is used for various purposes, such as customer segmentation, personalized marketing campaigns, and predictive analytics. By analyzing customer data, machine learning algorithms can identify distinct customer segments based on demographics, behavior, or preferences. This segmentation allows marketers to tailor their messages and offers to specific groups, increasing the effectiveness of marketing campaigns.
Personalized marketing campaigns are another significant application of machine learning in marketing. By analyzing individual customer data, including browsing history, purchase patterns, and social media activity, machine learning algorithms can generate personalized recommendations, advertisements, or product suggestions. This level of personalization enhances customer engagement and increases the likelihood of conversions.
Predictive analytics is a crucial aspect of marketing and customer analytics. Machine learning algorithms can analyze historical data and identify patterns or trends that can be used to predict future customer behavior, such as likelihood to churn, purchase probability, or response to marketing campaigns. These predictions enable marketers to proactively target customers with relevant offers or interventions to maximize customer retention and increase sales.
4. Natural Language Processing (NLP):
NLP is a subfield of machine learning that focuses on the interaction between computers and human language. It has applications in sentiment analysis, language translation, text summarization, chatbots, and voice assistants. NLP algorithms can extract insights from unstructured textual data, enabling organizations to understand customer feedback, analyze social media sentiment, and automate customer support processes.
5. Autonomous Vehicles:
Machine learning is at the core of developing autonomous vehicles. Through the analysis of sensor data, including cameras, LIDAR, and radar, machine learning algorithms can help vehicles perceive their surroundings, detect objects, and make real-time decisions to navigate safely. Machine learning techniques, such as computer vision and deep learning, are instrumental in advancing autonomous driving technologies.
6. Image and Speech Recognition:
Machine learning has revolutionized the fields of image and speech recognition by enabling computers to understand and interpret visual and auditory data. Image recognition involves training algorithms to identify and categorize objects, scenes, or patterns within images. By analyzing large datasets of labeled images, machine learning models can learn to recognize objects, detect faces, and even understand complex scenes. This technology has numerous applications, ranging from facial recognition in security systems to object recognition in autonomous vehicles.
Similarly, speech recognition utilizes machine learning algorithms to convert spoken language into written text. By training on vast amounts of audio data, machine learning models can learn to recognize and transcribe speech accurately. This technology powers virtual assistants, voice-controlled devices, and transcription services, making human-computer interaction more intuitive and accessible.
The success of image and speech recognition in machine learning is largely attributed to deep learning techniques, such as convolutional neural networks (CNNs) for image recognition and recurrent neural networks (RNNs) for speech recognition. These neural network architectures have shown remarkable performance in analyzing complex visual and auditory data, achieving state-of-the-art results in various recognition tasks.
Moreover, machine learning-based image and speech recognition systems continue to improve through techniques like transfer learning, which enables models to leverage knowledge gained from one task and apply it to another, and adversarial training, which enhances the robustness and reliability of recognition models against adversarial attacks.
7. Cybersecurity:
Machine learning is making significant contributions to the field of cybersecurity by enhancing threat detection, prevention, and response capabilities. By analyzing vast amounts of data and learning from patterns and anomalies, machine learning algorithms can identify and predict potential cyber threats in real-time. These algorithms can detect abnormal network behavior, identify malware, and classify known and unknown threats. Additionally, machine learning enables the development of intelligent systems that can adapt and evolve to new and emerging threats. By leveraging machine learning in cybersecurity, organizations can enhance their ability to detect and mitigate cyber attacks, improving overall security posture and safeguarding sensitive information.
The scope of machine learning is vast and ever-expanding, offering opportunities for innovation and advancements across industries. From healthcare to finance, marketing to autonomous vehicles, machine learning is transforming the way we solve complex problems, make predictions, and derive insights from data. As technology continues to evolve, the possibilities for machine learning applications are boundless. Embracing this powerful technology can unlock new avenues for growth, efficiency, and better decision-making in the digital era.