The Future of Machine Learning
Explore machine learning, the future, trends, AI, technology, innovation, applications, challenges, advancements, predictions, and data science.
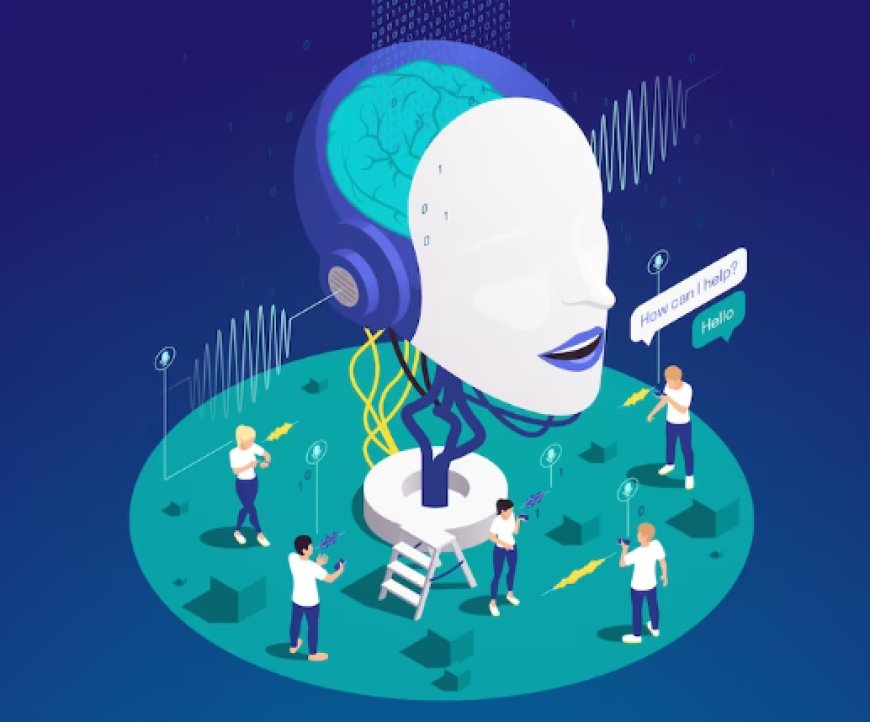
The future of machine learning holds the promise of reshaping industries, revolutionizing technology, and transforming our everyday lives. As this dynamic field continues to evolve, it presents us with a landscape of opportunities, challenges, and ethical considerations that will shape the way we harness artificial intelligence to address complex problems and improve our world. In this discussion, we will delve into the potential trajectories, innovations, and obstacles that lie ahead on the exciting journey of machine learning's future.
Current State of Machine Learning
The current state of Machine Learning (ML) is characterized by remarkable growth and widespread adoption across various industries and domains. ML is a subset of artificial intelligence (AI) that focuses on the development of algorithms and models capable of learning and making predictions or decisions based on data. Today, ML techniques and algorithms have become an integral part of our daily lives, often operating behind the scenes in applications ranging from recommendation systems on streaming platforms to autonomous vehicles and medical diagnostics.
In terms of techniques and algorithms, the field of ML has seen significant advancements. Deep learning, a subset of ML, has gained substantial prominence. Deep neural networks, inspired by the structure of the human brain, have demonstrated exceptional capabilities in tasks such as image recognition, natural language processing, and even playing complex games like Go. These deep learning models, such as convolutional neural networks (CNNs) and recurrent neural networks (RNNs), have set the stage for breakthroughs in areas like computer vision, speech recognition, and language translation.
Moreover, the availability of large-scale datasets and the computational power to process them have played a pivotal role in the current state of ML. The advent of cloud computing and specialized hardware, such as graphics processing units (GPUs), has enabled researchers and practitioners to train increasingly complex models. This has led to improved accuracy and performance across a wide range of applications, from self-driving cars to personalized healthcare.
Challenges in Machine Learning
-
Data Privacy and Ethical Concerns: Machine learning models heavily rely on large datasets, and handling sensitive or personal data raises significant privacy and ethical concerns. Ensuring that data is used responsibly, protecting individuals' privacy, and complying with data protection regulations (e.g., GDPR) are ongoing challenges.
-
Bias and Fairness in ML Algorithms: Bias can creep into machine learning algorithms, leading to unfair or discriminatory outcomes, especially when training data reflects historical biases. Addressing and mitigating bias in algorithms to ensure fairness is a critical challenge in machine learning.
-
Scalability and Computational Limitations: As machine learning models become more complex, they require substantial computational resources. Scaling up infrastructure to support large-scale training and deployment can be costly and technically challenging.
-
Interpretability and Transparency Issues: Many advanced machine learning models, like deep neural networks, are often seen as "black boxes" because they lack transparency. Understanding how these models arrive at decisions is essential for their adoption in critical applications such as healthcare and finance.
-
Data Quality and Availability: Access to high-quality data can be a bottleneck in machine learning. Garbage in, garbage out (GIGO) is a common problem where poor-quality data can lead to inaccurate or unreliable models. Acquiring, cleaning, and maintaining good-quality data is an ongoing challenge.
-
Regulatory and Legal Challenges: The regulatory landscape for machine learning is evolving. Compliance with regulations like GDPR, HIPAA (healthcare), or industry-specific standards can be complex and requires continuous monitoring and adaptation.
The Future of Machine Learning
The future of machine learning (ML) is a topic of significant interest and importance in today's rapidly evolving technological landscape. Here are some brief explanations about key aspects of this topic:
-
Continual Advancements in ML Algorithms: ML algorithms are constantly evolving, with a focus on improving accuracy, efficiency, and scalability. Future developments may include more advanced neural networks, reinforcement learning techniques, and innovative unsupervised learning methods, enabling machines to learn and adapt in more sophisticated ways.
-
Expanding Applications: Machine learning is finding applications in nearly every industry, from healthcare and finance to transportation and entertainment. In the future, we can expect ML to play an even larger role in these domains, leading to breakthroughs in areas like personalized medicine, autonomous vehicles, and more advanced recommendation systems.
-
Ethical Concerns and Fairness: As ML becomes more ingrained in society, there's a growing emphasis on addressing ethical concerns. The future of ML includes efforts to combat biases in algorithms, ensure fairness in decision-making, and establish responsible AI development practices to mitigate potential negative consequences.
-
Democratization of ML: The future promises increased accessibility to ML tools and techniques through no-code/low-code platforms and education initiatives. This democratization will empower a wider range of individuals and organizations to harness the power of machine learning for various purposes.
-
Quantum Computing's Impact: Quantum computing is expected to revolutionize ML by solving complex problems at speeds unattainable by classical computers. Quantum machine learning and quantum-enhanced algorithms have the potential to significantly accelerate ML advancements.
Challenges Ahead
As machine learning continues to evolve and play an increasingly prominent role in various aspects of our lives, several challenges loom on the horizon that must be addressed to ensure its responsible and sustainable development.
Data Quality and Availability: One of the primary challenges facing the future of machine learning is the quality and availability of data. While ML models heavily depend on large datasets for training and generalization, the data must be accurate, diverse, and free from biases. As the scale of data collection grows, ensuring its quality becomes more complex. Moreover, in some domains, such as healthcare or finance, access to high-quality data can be restricted due to privacy concerns or regulations. Balancing the need for data with privacy and ethical considerations is a persistent challenge.
Regulatory and Legal Challenges: The regulatory landscape surrounding machine learning is continually evolving. Issues related to data privacy, algorithmic transparency, and ethical AI are at the forefront of discussions. Governments and regulatory bodies are working to create guidelines and laws that strike a balance between fostering innovation and protecting individuals' rights and societal interests. Navigating this evolving regulatory environment while maintaining innovation will be a significant challenge for ML developers and organizations.
Human-Machine Collaboration and Augmentation: As AI and ML systems become more capable, there is a growing need to define the boundaries of human-machine collaboration. Determining when and how machines should assist humans, particularly in high-stakes decision-making contexts, raises complex ethical and practical questions. Striking the right balance to ensure that AI and humans complement each other effectively and ethically is an ongoing challenge.
The future of machine learning holds immense promise and potential. With ongoing advancements in algorithms, expanding applications across industries, and a growing emphasis on ethical development, machine learning is set to revolutionize the way we live and work. However, challenges related to data, ethics, and collaboration between humans and machines must be carefully navigated to ensure a positive and sustainable future for this transformative technology. The future of machine learning is not only exciting but also calls for responsible innovation to maximize its benefits while minimizing risks.