Machine Learning in Finance: Predictive Analytics and Risk Assessment
Explore the integration of machine learning in finance, uncovering how predictive analytics and risk assessment bolster financial decision-making
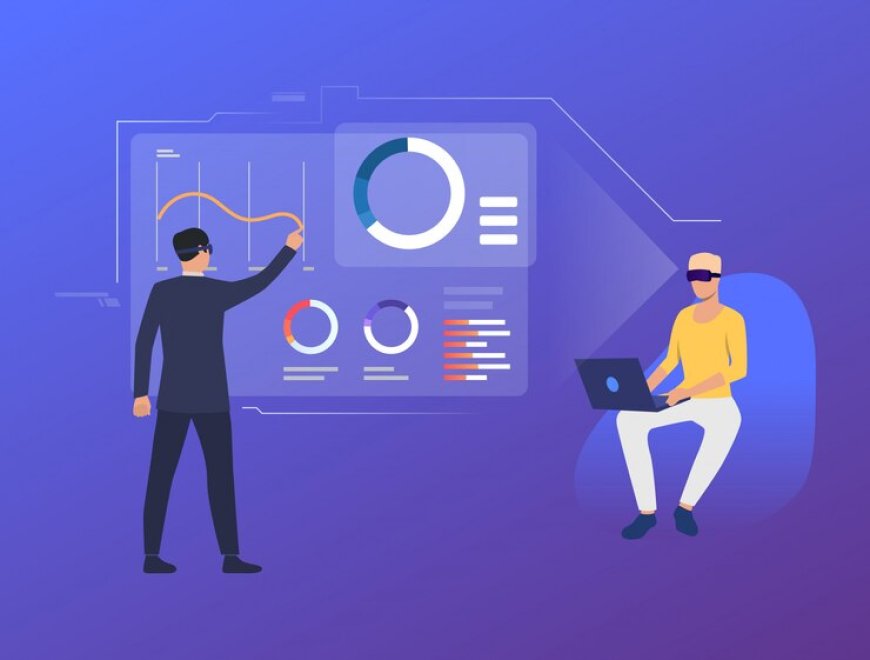
Machine learning has had a significant impact on a wide range of industries, and the world of finance is no different. we will take a closer look at the current situation of machine learning in finance. We'll explore the challenges it encounters and the methods used to address them. Additionally, we'll offer insights into common questions about this fascinating field.
Machine learning, a subset of artificial intelligence, has found its way into the heart of the financial sector, revolutionizing the way financial institutions operate. By harnessing the power of data-driven insights and predictive analytics, it has brought about significant changes in various aspects of finance. In this article, we will delve deeper into the current state of machine learning in finance, examining its applications, benefits, challenges, and the strategies employed to overcome these challenges. Let's embark on a journey to explore the dynamic landscape of machine learning in the realm of finance.
Machine Learning Applied Areas in Finance
The application of machine learning in the financial industry is broad and impactful. Financial organizations have embraced this technology to enhance their operations in several key areas:
Fraud Detection: Machine learning algorithms are adept at spotting unusual patterns and anomalies in financial transactions. They can quickly identify potentially fraudulent activities and trigger alerts, helping to protect both institutions and their customers from financial fraud.
Algorithmic Trading: Machine learning plays a significant role in algorithmic trading, where complex algorithms analyze market data and execute trades at high speeds. These algorithms can identify trading opportunities, optimize trading strategies, and adapt to changing market conditions in real-time.
Credit Risk Assessment: Assessing credit risk is a critical function in finance, and machine learning models excel in this area. They can analyze vast datasets to evaluate the creditworthiness of individuals and businesses, helping financial institutions make more informed lending decisions.
Customer Service Chatbots: Machine learning-powered chatbots provide instant and personalized customer support. They can handle routine inquiries, process transactions, and offer guidance, enhancing the overall customer experience while reducing the workload on human customer service agents.
Machine learning brings a host of benefits to the financial sector, contributing to its widespread adoption:
Real-Time Risk Assessment: Machine learning models can continuously monitor financial activities and assess risks in real-time. This proactive approach allows financial institutions to respond swiftly to potential issues, minimizing losses and protecting their assets.
Fraud Detection: The ability to detect fraudulent transactions quickly and accurately is a significant advantage. Machine learning algorithms can identify unusual patterns and behaviors that may indicate fraud, significantly reducing financial losses due to fraudulent activities.
Personalized Financial Recommendations: Machine learning can analyze customer data and behavior to provide personalized financial recommendations. This not only enhances the customer experience but also helps individuals make more informed financial decisions.
Automation of Routine Tasks: Machine learning automates routine and repetitive tasks, reducing the need for manual intervention. This automation not only improves efficiency but also lowers operational costs for financial institutions.
Challenges in Implementing Machine Learning in Finance
While the potential of machine learning in finance is extensive, there are significant challenges that need to be addressed:
1. Data Quality and Quantity: The accuracy and reliability of machine learning models in finance depend heavily on the quality and quantity of the data they are trained on. Financial data is often unstructured, and noisy, and can contain errors or missing information. These issues can make it difficult to extract meaningful insights. Implementing data cleansing and validation processes is crucial to mitigate this challenge and ensure that the data used for training is of high quality.
2. Regulatory Compliance: Financial institutions operate in a heavily regulated environment, and compliance with these regulations is of utmost importance. Machine learning models must adhere to various financial regulations, which can be complex and subject to frequent changes. Tailored compliance solutions specifically designed for machine learning applications are necessary to address this challenge effectively and ensure that models meet the required standards.
3. Model Interpretability: Interpreting machine learning models, especially in financial contexts, can be a complex task. Financial professionals and regulators often need to understand the decision-making processes of these models, particularly in areas like customer credit assessments where transparency is critical. Explainable AI (XAI) techniques are essential for providing clarity and insights into how machine learning models arrive at their decisions, making them more interpretable and trustworthy.
What are all Hurdles in Machine Learning's Potential in Finance
What are the primary challenges when addressing the implementation of machine learning in finance? These challenges encompass data quality and quantity, regulatory compliance, and model interpretability. How can these challenges be effectively addressed to fully realize the potential of machine learning in the financial sector?
How to Overcome the above challenges?
Addressing these challenges is essential for harnessing the full potential of machine learning in finance while maintaining transparency, compliance, and the trust of stakeholders in the financial industry. To fully realize the potential of machine learning in finance.
Data Management: Improving data quality and quantity is foundational. This can be achieved through systematic data cleansing, validation, and integration. Financial organizations should consider investing in data management solutions that streamline these processes, significantly enhancing the accuracy and reliability of machine learning models. Moreover, they should establish data governance practices to maintain data quality over time.
Compliance Solutions: In the heavily regulated financial sector, specialized compliance solutions are a necessity. These solutions should be customized to meet the unique requirements of machine learning applications. They ensure that machine learning models remain transparent, interpretable, and in compliance with the constantly evolving financial regulations. Regular audits and updates of these compliance solutions are crucial to staying current with regulatory changes.
Explainable AI: Enhancing model interpretability is key. In addition to LIME and SHAP, organizations can explore other explainable AI techniques like feature importance analysis and model-agnostic explanations. These methods offer clear insights into how decisions are made by machine learning models, enhancing transparency and accountability. Financial professionals should be trained to understand these techniques and their applications.
Robust Validation and Testing: Rigorous validation and testing processes are vital to prevent overfitting and ensure model generalization. Techniques such as cross-validation and out-of-sample testing should be implemented to assess the model's performance under different scenarios and to avoid reliance on historical data alone.
Ethical Considerations: As machine learning models become more integrated into financial decision-making, ethical considerations must be a top priority. Organizations should establish clear guidelines for the ethical use of AI in finance to ensure fair and unbiased outcomes.
Continuous Monitoring: Ongoing monitoring of machine learning models is necessary to detect and address issues promptly. Anomalies and model drift should be monitored, and models should be retrained as needed to adapt to changing financial conditions and regulations.
While machine learning in finance faces challenges related to data, regulations, and model interpretability, it offers unparalleled advantages and opportunities. By implementing effective data governance, leveraging RegTech, and embracing model explainability techniques, financial institutions can overcome these challenges and harness the transformative power of machine learning. This integration will not only improve operational efficiency but also enhance customer experiences, making it a win-win for both the financial industry and its clients. Machine learning in finance is not merely a trend; it is the future of the industry.