Machine Learning in Cybersecurity: Protecting the Digital Frontier
Learn about the application of advanced algorithms for securing digital assets in the modern cyber landscape
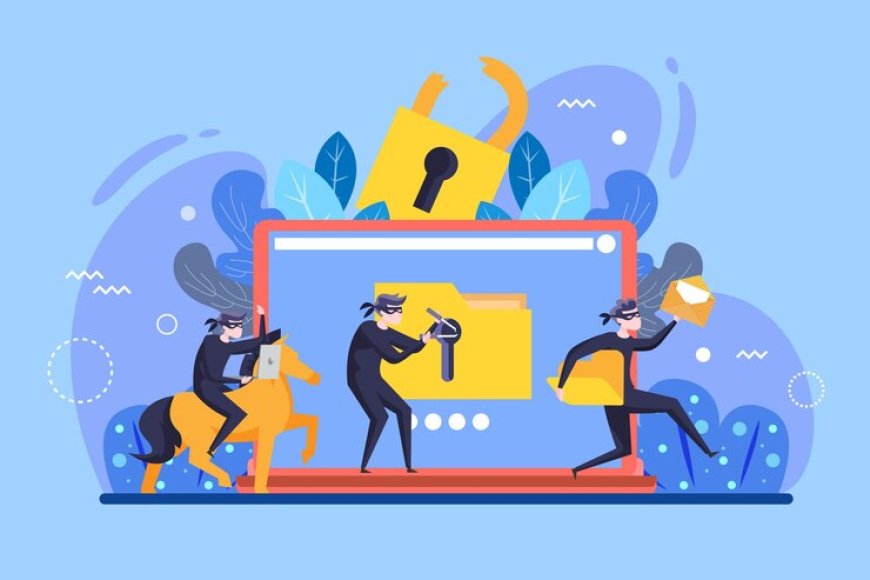
Machine learning is like a digital guardian that helps keep our online world safe. It's a smart technology that learns from past experiences and uses that knowledge to detect and prevent cyberattacks. Think of it as having a super-smart security system that can recognize suspicious behavior and stop hackers in their tracks. This technology plays a crucial role in the field of cybersecurity, which is all about protecting our digital frontier. Whether it's guarding our personal information, defending businesses from data breaches, or even shielding our national security, machine learning is a powerful ally in the battle against cyber threats.
Cybersecurity in machine learning refers to the application of machine learning techniques and technologies to enhance the security of digital systems and data. It involves using machine learning algorithms to detect and mitigate cyber threats such as malware, phishing attacks, and network intrusions. Machine learning models analyze vast amounts of data to identify patterns, anomalies, and potential vulnerabilities, enabling organizations to proactively defend against evolving threats and strengthen their overall cybersecurity posture. This intersection of cybersecurity and machine learning provides more adaptive and efficient security solutions in the face of an ever-changing threat landscape.
Businesses and individuals are heavily reliant on the Internet for communication, commerce, and data storage. As a result, cyber threats have become more pervasive and diverse. Attackers continuously devise new tactics to infiltrate networks, steal sensitive information, and disrupt critical services. Traditional signature-based security systems are no longer enough to combat these dynamic threats effectively.
Traditional security tools, such as firewalls and antivirus software, rely on predefined rules and known threat signatures. They struggle to adapt to new, previously unseen threats. Additionally, attackers employ advanced evasion techniques, making it challenging to detect their activities. This leaves organizations vulnerable to zero-day attacks, where exploits are used before security patches are available.
Understanding the Significance of Machine Learning in Cybersecurity
It is an era when data breaches, ransomware attacks, and other cyber threats are making headlines regularly, and the importance of machine learning in cybersecurity cannot be overstated. Here, we delve deeper into the significance of this technological advancement.
Adaptability:
Machine learning models can adapt to new threats without requiring constant updates. Traditional signature-based systems rely on databases of known threats, leaving organizations vulnerable to unknown attacks. Machine learning, on the other hand, can analyze new data and identify anomalies, making it a more adaptive and proactive solution.
Identifying Unknown Threats:
As cyber attackers continually develop new techniques, it's crucial to have a system that can detect previously unseen threats. Machine learning models excel in recognizing unusual patterns and behaviors in network traffic or user activity, thus helping to identify zero-day vulnerabilities.
Improved Accuracy:
Machine learning algorithms can reduce false positives by learning from past data and understanding the normal behavior of a system. This results in more accurate threat detection, allowing cybersecurity teams to focus their efforts on genuine threats rather than sifting through a sea of false alarms.
Predictive Capabilities:
Machine learning can predict potential security threats based on historical data. By analyzing user behavior and network activities, it can anticipate and mitigate threats before they escalate. This proactive approach is invaluable in the world of cybersecurity.
Addressing Challenges in Implementing Machine Learning in Cybersecurity
While the potential benefits of machine learning in cybersecurity are substantial, it's essential to recognize and address the challenges associated with its implementation:
Data Quality:
Machine learning models heavily depend on the quality of the data used for training. Inaccurate or biased data can lead to flawed models. It's crucial to ensure that the training data is representative of real-world scenarios and that it doesn't contain biases that could lead to false positives or negatives.
Adversarial Attacks:
Machine learning models can be vulnerable to adversarial attacks. These attacks involve deliberately manipulating data input to deceive the model and make it misclassify information. Security researchers and practitioners must work on robustifying machine learning models against such attacks.
Privacy Concerns:
Collecting and analyzing user data for security purposes can raise privacy concerns. Organizations must strike a balance between security and individual privacy rights. Implementing strong data protection measures and complying with relevant regulations is crucial.
Skill Gap:
Machine learning expertise is in high demand, and there's a significant shortage of professionals with the necessary skills. Organizations need to invest in training and development to ensure that their teams can effectively implement and manage machine learning-based cybersecurity solutions.
Integration Challenges:
Integrating machine learning into existing cybersecurity infrastructure can be complex. It requires a seamless connection between ML models and security systems, as well as the ability to act on ML-generated insights in real time.
Applications of Machine Learning in Cybersecurity
Machine learning's impact on cybersecurity extends to various applications, each playing a vital role in safeguarding digital assets. Here are some of the key applications:
Anomaly Detection:
Anomaly detection is a foundational application of machine learning in cybersecurity. ML models learn the normal behavior of network traffic, system activities, and user actions. When deviations from the norm occur, it raises suspicion and triggers alerts. This approach is highly effective in identifying previously unknown threats and zero-day vulnerabilities.
Predictive Analysis:
Machine learning can analyze historical data to identify patterns and trends associated with cyber threats. By recognizing these patterns, it can predict potential threats. For example, if a particular combination of network events has preceded past data breaches, machine learning can flag similar occurrences in real time and allow cybersecurity professionals to act swiftly.
Threat Intelligence:
Cyber threat intelligence involves collecting and analyzing vast amounts of data from various sources to identify emerging threats and vulnerabilities. Machine learning can significantly enhance this process by sifting through large datasets, detecting correlations, and providing valuable insights into the evolving threat landscape. It helps organizations stay ahead of attackers by proactively addressing vulnerabilities.
Natural Language Processing (NLP):
NLP, a subfield of machine learning, plays a crucial role in email security and content analysis. It can assess the content and context of messages to detect phishing attempts, malicious attachments, or suspicious communication. By understanding the intent behind the text, NLP can separate legitimate messages from threats.
Machine learning is a powerful ally in the battle against cyber threats. Its ability to adapt and learn from new data and evolving attack techniques makes it a crucial component of modern cybersecurity strategies. Machine learning excels at identifying unknown threats, reducing false positives, and providing predictive insights that allow organizations to stay one step ahead of attackers.