The Impact of Data Science on Business Strategy
From informed decision-making to gaining a competitive edge, discover how businesses leverage data science for success.
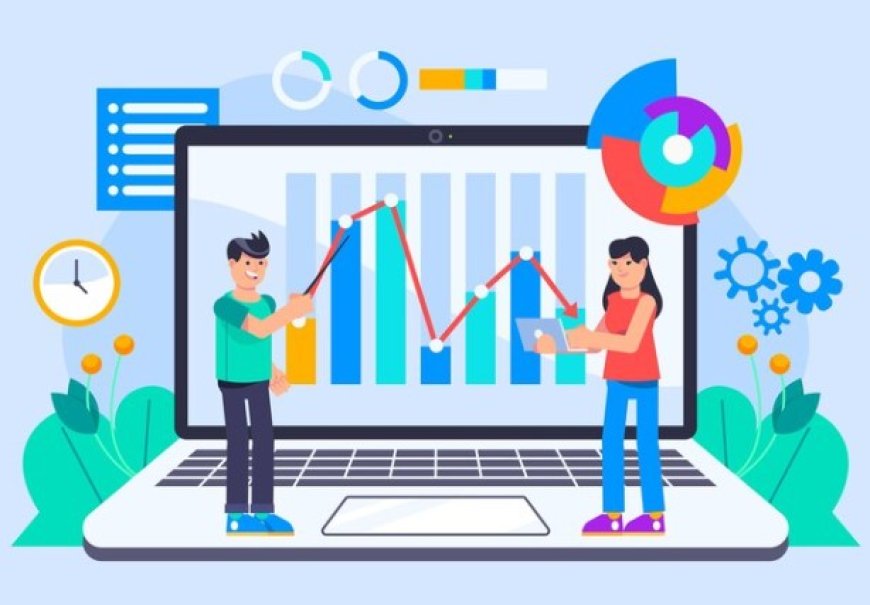
In recent years, the growing significance of data science has become increasingly evident in the business environment. As organizations accumulate vast amounts of data, the transformative role of data in decision-making processes has become a cornerstone of strategic planning and execution. The sheer volume and complexity of data generated today have led to a paradigm shift, where businesses are harnessing the power of data science to extract valuable perspectives and drive informed decision-making.
Data science plays a pivotal role in transforming raw data into actionable intelligence, enabling businesses to make decisions that are not only well-informed but also predictive in nature. This transformative capacity of data science is not confined to a specific industry; rather, it permeates various sectors, influencing how organizations operate, compete, and innovate.
Data Science and Its Core Principles
Data science is a multidisciplinary field that utilizes scientific methods, processes, algorithms, and systems to extract insights and knowledge from structured and unstructured data. At its core, data science combines expertise from statistics, mathematics, computer science, and domain-specific knowledge to analyze complex datasets, uncover patterns, and inform decision-making.
Core Principles of Data Science:
1. Data Collection: Gathering relevant and representative datasets from various sources.
2. Data Cleaning: Preprocessing and cleansing data to ensure accuracy and reliability.
3. Exploratory Data Analysis (EDA): Understanding the data through visualization and statistical analysis.
4. Feature Engineering: Selecting and transforming variables to enhance model performance.
5. Model Building: Employing statistical and machine learning models to derive insights.
6. Validation and Testing: Assessing model accuracy and generalizability.
7. Interpretation: Extracting meaningful insights and actionable information from the results.
Evolution of Data Science in Business Environments
The evolution of data science in business has been marked by a progression from traditional data analysis to a more sophisticated, strategic role. Initially focused on descriptive analytics, businesses used data to summarize and report past performance. Over time, the field expanded to include predictive analytics, allowing organizations to forecast future trends and outcomes.
In the last few years, the rise of prescriptive analytics has further elevated the impact of data science on business strategy. Prescriptive analytics not only predicts future scenarios but also recommends actions to optimize outcomes. This evolution reflects a shift from hindsight-based decision-making to a more forward-thinking, proactive approach.
Data science is now deeply integrated into various business functions, including marketing, finance, operations, and human resources. Its application spans industries, driving innovation, efficiency, and competitiveness.
Statistics on the Increasing Adoption of Data-Oriented Decision-Making in Organizations
1. Global Adoption Trends: A survey conducted across industries globally reveals that over 80% of organizations have embraced data-oriented decision-making as a strategic priority.
2. Business Impact: Organizations leveraging data science report improved business performance, with a noticeable increase in revenue and a reduction in operational costs.
3. Industry-Specific Cases: Examples from sectors such as e-commerce, healthcare, and finance showcase how data-driven decisions have led to more targeted marketing campaigns, enhanced patient care, and optimized financial strategies.
4. Technology Investment: Companies are increasingly investing in advanced analytics tools, machine learning platforms, and data science talent to stay competitive in an evolving market.
These statistics underscore the widespread recognition of data science as a catalyst for business success, encouraging organizations to leverage data-driven perspectives for strategic decision-making and gain a competitive edge in their respective industries.
Challenges Businesses Face in the Absence of Business Strategies
Businesses not implementing data-driven strategies face a number of obstacles that lower their overall performance and competitiveness in an era of plentiful data.
-
Limited Strategic Vision:
Without data-driven insights, businesses may lack a comprehensive understanding of market trends, customer behaviors, and emerging opportunities, limiting their ability to develop a forward-looking strategic vision.
-
Ineffective Resource Allocation:
The absence of data-driven decision-making may result in suboptimal allocation of resources. Businesses may struggle to identify high-impact areas for investment, leading to inefficient use of time, money, and manpower.
-
Reduced Competitiveness:
In industries where competitors harness data for strategic advantage, businesses without data-driven strategies risk falling behind. They may miss out on opportunities to innovate, optimize processes, and deliver superior products or services.
Examples of Missed Opportunities or Suboptimal Outcomes Due to Insufficient Data Utilization
-
Marketing Inefficiencies:
A business relying on traditional marketing strategies may miss opportunities to target specific demographics effectively. Data-driven marketing enables personalized campaigns, improving engagement and conversion rates.
-
Inventory Management Challenges:
Without data-driven insights, businesses may struggle with inventory management, leading to overstock or stockouts. Data science helps optimize inventory levels based on demand forecasting.
-
Customer Experience Gaps:
Businesses not leveraging customer data may fail to understand preferences, resulting in subpar customer experiences. Data-driven insights enhance personalization, leading to improved customer satisfaction and loyalty.
-
Risk Mitigation Oversights:
In sectors like finance or insurance, businesses without robust data-driven risk analysis may overlook potential threats, leading to financial losses. Data science aids in identifying and mitigating risks proactively.
Three key questions surface as companies go around a data oriented era:
In what ways can companies use data science to improve the quality of their strategic decision-making? Which particular areas of corporate strategy is data science most promising to transform? Furthermore, in what ways does the incorporation of data science offer a significant edge over competitors in the ever-changing world of contemporary business?
Now for the solution:
A. Enhancing Decision-Making
At the forefront of data science's impact lies its pivotal role in enhancing decision-making processes. By analyzing vast datasets, data science provides organizations with actionable insights crucial for informed decision-making. The ability to distill patterns and trends from complex data minimizes uncertainty surrounding strategic choices. For instance, predictive analytics can forecast market trends, allowing businesses to proactively adjust their strategies. In doing so, data science not only minimizes uncertainty but also significantly improves the accuracy of strategic decisions. Real-time analysis and machine learning algorithms contribute to a dynamic decision-making environment, empowering businesses to navigate complexities with a level of precision and foresight that traditional methods often lack.
B. Customer Experiences
Businesses are looking more and more on data science for strategy. Organizations can find insights that align with individual preferences by utilizing data science to explore the complex patterns of customer behavior and preferences. By utilizing advanced analytics and machine learning algorithms, companies are able to carefully examine large datasets in order to identify complex consumer preferences. The application of data-driven personalization is demonstrated by a number of case studies, which demonstrate how this analytical skill translates into tailored strategy. These tactics, whether used in retail, streaming services, or e-commerce, have repeatedly shown to have a significant impact on client loyalty and happiness. Businesses can foster enduring customer loyalty and improve customer engagement by providing personalized experiences that correspond with individual preferences. This allows them to take the lead in an increasingly customer-centric market.
C. Optimizing Operations
Data science serves as a powerful catalyst for operational optimization, streamlining processes and enhancing efficiency across various business domains. By leveraging advanced analytics and machine learning, organizations can derive actionable insights that lead to more efficient operations. For instance, in supply chain management, data science enables real-time visibility into the entire supply chain, improving forecasting accuracy and reducing excess inventory. Businesses can strategically allocate resources based on data-driven insights, optimizing workforce productivity and resource utilization. This transformative application of data science in operations is exemplified by industry leaders who have successfully harnessed data to enhance supply chain resilience, reduce costs, and create a leaner, more responsive operational framework.
D. Predictive Analytics for Future Planning
Predictive analytics, a cornerstone of data science, empowers businesses to go through the future with foresight. By utilizing historical data and sophisticated algorithms, organizations can forecast trends, anticipate market changes, and identify potential challenges before they unfold. This capability provides a strategic advantage, enabling proactive planning based on insights derived from predictive analytics. Rather than merely reacting to events, businesses can formulate robust strategies, allocate resources effectively, and position themselves to capitalize on emerging opportunities. In essence, predictive analytics serves as a strategic compass, guiding businesses toward a future where informed decisions are made with confidence, resilience, and a keen understanding of the dynamic forces shaping their industries.
E. Competitive Edge
Businesses wielding data science as a strategic ally gain a formidable competitive edge. By scrutinizing vast datasets, organizations can extract invaluable insights that inform strategic decisions, enhancing their ability to stay agile in dynamic markets. The integration of data science fosters operational efficiency, targeted marketing, and innovation, positioning companies to outperform competitors.
Success stories abound, exemplifying the transformative impact of data science. Companies leveraging predictive analytics for market trends anticipate shifts, enabling them to adapt swiftly and proactively. Personalized customer experiences, fueled by data-driven strategies, foster brand loyalty and distinguish businesses in crowded markets. The strategic integration of data science is not merely a technological augmentation but a paradigm shift, empowering businesses to lead with foresight, resilience, and a distinct competitive advantage.
In conclusion, it's clear that data science has profoundly changed how businesses make decisions and stay competitive. Whether it's helping with choices or giving an advantage over rivals, data science is crucial for today's companies. Thinking about what we've discussed, it's easy to see that using data science isn't just a one-time thing; it keeps evolving.
As we look ahead, the progress in data science is exciting. New technologies like artificial intelligence and machine learning will keep making decision-making even better for businesses. This means companies can handle challenges more accurately in the future.