10 Ways of Data Science Applications
Explore the diverse landscape of data science applications with our guide. From revolutionizing healthcare to reshaping industries, discover the real-world
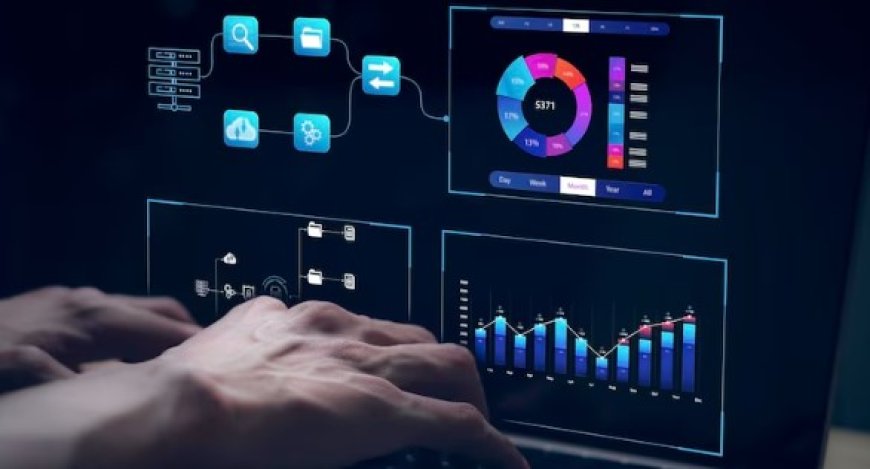
A small retail company faces consumer engagement and inventory management issues in a changing business environment. Recognizing the need for a strategic shift, the corporation seeks meaningful insights through data science. Rather than adopting a generic approach, they use specialized data science applications to solve specific pain points. Customer interactions become more individualized, resulting in higher satisfaction and loyalty. Inventory management improves significantly as a result of the ideal stocking levels and fewer instances of overstock or shortages. This practical application of data science illustrates its practical integration into the company's day-to-day operations, diverging from standard strategies and showcasing its effectiveness in resolving real-world business challenges.
How Data is Revolutionizing Business
Today's business world is changing, moving away from traditional ways of doing things to a new focus on using data effectively. This shift recognizes that harnessing data can give organizations the power to make smart decisions, stay competitive, and quickly adapt to a constantly changing market. Let's explore this transformation, looking at how strategies for integrating data are replacing traditional methods and driving a new era of decision-making.
Current Business Environment
Companies in today's hectic business environment must deal with massive amounts of data from many sources such as client interactions, market trends, and day-to-day operations. Extracting useful insights from this data has become critical. Traditional business approaches that rely on history and intuition are struggling to stay up in this data-rich world.
Evolving Data Integration Strategies
Data integration is now a key part of creating a flexible and responsive organizational structure. Businesses are investing in advanced technologies like cloud computing, analytics, and machine learning to bring together data from different sources. This helps create a complete picture of a company's data and a deeper understanding of how the business works.
Key Changes in the Paradigm
Data-Driven Decision-Making
Old ways of making decisions, often based on personal judgments, are being replaced by data-driven decision-making. Companies are using analytics to get practical insights, allowing leaders to base decisions on solid evidence rather than guesses. This change is making decision-making more objective and efficient.
Real-Time Adaptability
The ability to integrate data in real-time lets companies respond quickly to market shifts and customer needs. By keeping an eye on key performance indicators as they happen, businesses can adjust their strategies on the spot, staying nimble in a constantly changing business environment.
Improved Customer Experience
Data-driven approaches are transforming how companies understand and connect with their customers. Using data insights for personalized interactions is becoming a vital part of effective customer relationship management. This, in turn, boosts customer satisfaction and loyalty.
Innovation and Flexibility
Organizations that use data are better at fostering innovation. By using insights from integrated data, companies can spot emerging trends, find new opportunities, and quickly adjust to take advantage of changes in the market.
Traditional Challenges and Limitations
Limitations
Limited Insights and Informed Decision-Making: Traditional business models often rely on historical data and intuition for decision-making. This can lead to limited insights into current market trends, customer behaviors, and emerging opportunities. Without data science applications, businesses may struggle to make informed and strategic decisions.
Dependency on Manual Processes: Many traditional models heavily rely on manual processes for tasks such as data collection, analysis, and reporting. This not only consumes time but also increases the likelihood of errors. Data science can automate these processes, allowing for more accurate and efficient operations.
Inability to Harness Big Data: With the increasing volume of data in the digital age, traditional business models may find it challenging to handle and extract meaningful insights from big data. Data science applications, such as machine learning algorithms, enable businesses to analyze vast amounts of data, uncover patterns, and derive valuable insights.
Lack of Personalization: Without data-driven insights, traditional models may struggle to personalize customer experiences. Data science allows businesses to understand individual preferences and behavior, facilitating the delivery of personalized products, services, and marketing strategies.
Inefficient Resource Allocation: Traditional models may face difficulties in optimizing resource allocation due to a lack of data-driven forecasting. This can lead to overinvestment in certain areas and underinvestment in others. Data science can provide predictive analytics to optimize resource allocation and enhance overall efficiency.
Challenges
Inefficient Decision-Making: Traditional models cannot often leverage real-time data and advanced analytics for decision-making.
Slow Decision-Making Processes: Without data science applications, decision-making processes can be slow and hindered by a lack of timely information.
Lack of Agility: Traditional models may have rigid processes that are resistant to change, making it challenging to adapt to rapidly evolving market conditions.
Slow Response to Market Changes: Without agile methodologies supported by data science, businesses may struggle to respond quickly to shifts in customer preferences, competition, or economic factors.
Missed Opportunities: Traditional models may miss out on identifying and capitalizing on emerging market trends without the analytical capabilities provided by data science.
Limited Innovation: Lack of data-driven insights can stifle innovation, preventing businesses from exploring new products, services, or business models that could lead to growth.
Difficulty in Forecasting and Planning: Traditional models may rely on simplistic forecasting methods, leading to inaccurate predictions and suboptimal planning.
Risk of Overlooking Key Metrics: Without data science, businesses may overlook crucial metrics and key performance indicators necessary for effective planning and performance evaluation.
Competitive Disadvantage: In industries where competitors leverage data science for strategic decision-making, businesses without such capabilities may face a competitive disadvantage.
Limited Customer Understanding: Traditional models may struggle to deeply understand customer behavior, preferences, and needs, hindering the development of competitive advantages based on customer-centric strategies.
10 Transformative Ways Data Science Reshapes Corporates
1. Informed Decision-Making Through Predictive Analytics:
Data science transforms decision-making by harnessing predictive analytics. Through a comprehensive analysis of historical and real-time data, businesses gain insights that guide strategic planning. This approach replaces uncertainty with precision, exemplified by optimizing marketing strategies through predictive models, and efficiently allocating resources to maximize returns.
2. Operational Optimization Through Data-Driven Efficiency:
Data science plays a critical role in optimizing operations by identifying and streamlining processes. It enables businesses to enhance efficiency by addressing bottlenecks and ensuring resource allocation is well-optimized. Real-time monitoring becomes feasible, allowing for immediate adjustments and continuous improvement.
3. Personalized Customer Experiences Through Data Analysis:
Customer relationship management evolves with data science, enabling businesses to create personalized experiences that foster loyalty. Data-driven insights inform tailored marketing strategies, dynamic pricing models, and a nuanced understanding of customer preferences, contributing to sustained customer satisfaction and loyalty.
4. Fraud Detection and Risk Mitigation:
Data science proves indispensable in detecting and preventing fraud, employing advanced analytics for proactive risk management. Enhanced security measures, guided by data insights, safeguard organizations from potential threats. Furthermore, data science ensures regulatory compliance, reducing the risk of legal consequences.
5. Employee Productivity and Engagement:
Data science applications enhance talent management, influencing employee productivity and satisfaction. Decision-making, from recruitment to engagement strategies, is informed by data-driven insights. Targeted interventions based on data analysis further improve employee satisfaction, contributing to enhanced performance.
6. Market Intelligence and Competitive Advantage:
Businesses gain valuable market insights through data science, shaping strategic decisions. Continuous market analysis allows for quick adaptation, providing a competitive edge. Understanding customer behavior through data facilitates the development of customer-centric strategies.
7. Supply Chain Optimization Through Data Analytics:
Data science optimizes supply chain management, improving inventory management and reducing costs. Analytics aids in predicting equipment failures, facilitating proactive maintenance, and minimizing disruptions in the supply chain.
8. Strategic Resource Allocation with Advanced Analytics:
Data-driven insights impact strategic resource allocation, optimizing budgeting for maximum return on investment. Advanced analytics assist in identifying and mitigating potential risks associated with resource allocation decisions. Real-time data analysis allows for agile resource management, adapting strategies based on market conditions.
9. Improving Product and Service Innovation:
Data science fosters innovation in product development and services. By analyzing consumer insights, businesses identify market needs and create solutions aligned with customer preferences. Rapid prototyping, facilitated by data analysis, reduces time-to-market for new products, fostering an iterative approach to development.
10. Regulatory Compliance and Data Governance:
Ensuring regulatory compliance and ethical data governance is imperative in the data-driven era. Data science applications aid in ethical data handling, creating audit trails for transparency and accountability. Organizations can adapt quickly to changes in regulations, maintaining ongoing compliance and effective risk management.
In conclusion, the blog provides a comprehensive overview of how data science applications are reshaping corporate strategies. It outlines the transformative impact on decision-making, operational efficiency, and customer experiences. The blog highlights the strategic role of data science in fraud detection, risk mitigation, talent management, and market intelligence. Supply chain optimization, strategic resource allocation, and dynamic product innovation are also explored as key areas influenced by data science.