Data Science Course Accreditation: Path to Success
Explore the significance of data science course accreditation as the path to success. Discover the benefits and criteria while choosing accredited programs
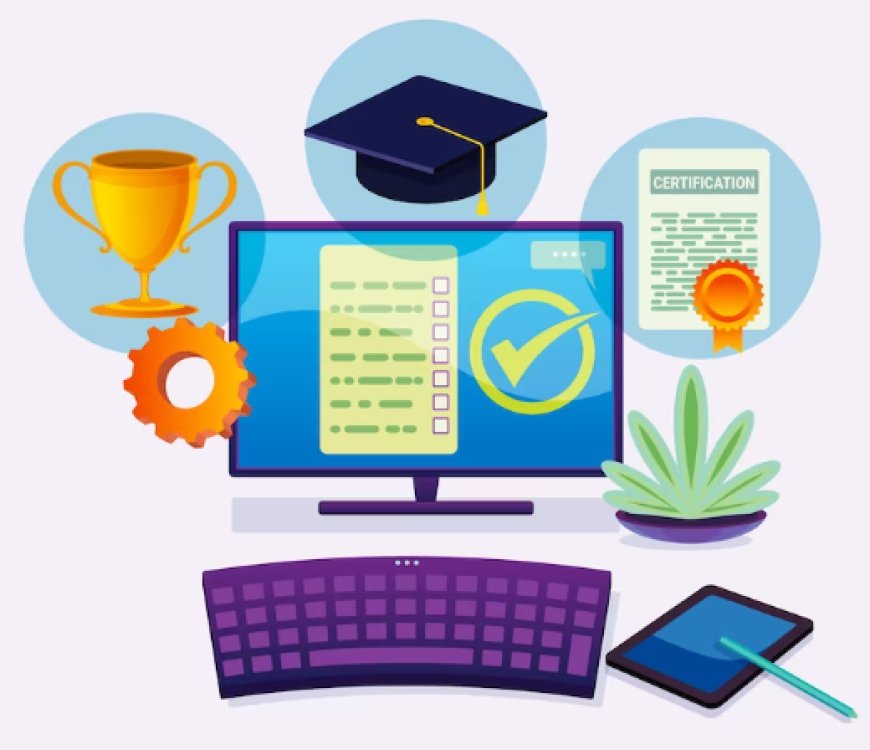
The demand for data science professionals is on the rise, and it is essential to have reliable and high-quality education in this field. Accreditation plays a critical role in ensuring that data science courses meet industry standards and provide a valuable educational experience. In this blog, we will explore the significance of accreditation in data science courses, the challenges it presents, and the benefits it brings.
The field of data science has experienced tremendous growth in recent years. Organizations in various industries are increasingly relying on data-driven decision-making, leading to a surge in the demand for data scientists. Consequently, educational institutions have responded by offering a wide range of data science courses and programs to meet this demand.
While the increase in data science courses is a positive development, it also presents challenges for both students and employers. With numerous options available, it can be difficult to identify educational programs that truly prepare students for careers in data science. This is where accreditation becomes vital.
The diversity of data science courses can lead to complications. It's important to note that not all programs are equal, and some may not offer the thorough education and training needed for success in the field. Without a standardized way to evaluate and ensure the quality of these programs, students may end up investing time and money in courses that don't provide the skills and knowledge they were promised. This can create challenges for employers when assessing the qualifications of potential job candidates. Accreditation plays a crucial role in indicating the quality of education a candidate has received, making it simpler for employers to identify well-prepared data science professionals.
Considering the intricate nature of data science education and the challenges it poses, it's important to address the following questions:
1. What is accreditation, and why is it important for data science courses?
Accreditation is a voluntary quality assurance process that educational institutions and programs undergo to demonstrate that they meet certain predetermined standards. In the context of data science courses, accreditation is essential for several reasons:
Quality Assurance: Through its stringent evaluation processes, accreditation serves as a crucial quality benchmark for data science programs. By adhering to recognized standards, accredited programs ensure that students receive an education that meets or exceeds industry expectations. This quality assurance instills confidence in students and employers, assuring them that graduates possess the necessary skills and knowledge to excel in the data science field.
Credibility: The mark of accreditation enhances the credibility of data science programs, fostering trust among students, employers, and the industry. In a competitive job market where the value of qualifications and skills is paramount, accredited programs stand out as reliable and reputable sources of education, attracting top talent and gaining the confidence of prospective employers.
Consistency: Accreditation promotes uniformity and consistency in the curriculum of data science programs, ensuring that students receive a standardized education that covers essential topics and skills. This consistency fosters a level playing field for students, regardless of the institution they attend, and equips them with a robust foundation in data science principles, methodologies, and practical applications.
Industry Relevance: Accreditation standards often integrate input from industry experts, guaranteeing that the curriculum remains pertinent and up-to-date with the latest advancements and trends in data science. By incorporating industry insights, accredited programs equip students with the knowledge and skills needed to tackle real-world challenges, enhancing their marketability and adaptability in the quickly changing data science environment.
Access to Financial Aid: Accredited data science programs are more likely to be eligible for various forms of financial aid, scholarships, and grants. This accessibility to financial resources makes education more affordable and attainable for a broader spectrum of students, promoting diversity and inclusivity within the field of data science. By removing financial barriers, accreditation fosters equal opportunities for students to pursue their academic and professional aspirations in data science, regardless of their economic backgrounds.
2. Accreditation Processes in Data Science Education:
Self-Assessment: Educational institutions seeking accreditation begin by conducting a thorough self-assessment, reviewing their programs, faculty qualifications, resources, and other relevant aspects.
Application: The institution submits an application to the accrediting body, expressing its intent to undergo the accreditation process.
Site Visit: Accrediting bodies may conduct site visits to assess the institution's facilities, and resources, and meet with faculty and students.
Review and Evaluation: The accrediting body reviews the institution's self-assessment, site visit reports, and other documentation to evaluate whether it meets the accreditation standards.
Decision: After a thorough review, the accrediting body makes a decision regarding accreditation. If accredited, the institution is expected to maintain ongoing compliance with the standards.
Periodic Review: Accreditation is not a one-time event; institutions are subject to periodic reviews to ensure they continue to meet the standards.
In the case of data science courses, accrediting bodies often consider factors such as the curriculum's comprehensiveness, the qualifications of faculty, the availability of relevant resources, and alignment with industry needs.
3. Benefits of Accredited Data Science Courses for Students:
Students who choose accredited data science courses enjoy several advantages:
Quality Assurance: Accredited programs are more likely to provide a high-quality education, ensuring that students acquire the skills and knowledge necessary to excel in the field.
Credibility: An accredited degree or certificate is more likely to be recognized and respected by employers and other institutions.
Access to Financial Aid: Accredited programs are often eligible for government-funded financial aid, scholarships, and grants, making education more affordable.
Networking Opportunities: Accredited programs may offer students access to industry events, conferences, and networks that can facilitate career advancement.
Employability: Graduates of accredited programs are generally more attractive to employers, as they can trust that the education received aligns with industry standards.
4. Accreditation's Role in Identifying Qualified Data Science Professionals:
For employers in search of qualified data science professionals, accreditation serves as a valuable filter in the hiring process. It helps in the following ways:
Quality Assurance: Accredited programs are more likely to produce graduates with the right skills and knowledge for the job.
Simplifies Hiring: Accreditation makes it easier for employers to identify candidates who have received a standardized, quality education.
Industry Trust: Hiring graduates of accredited programs instill confidence in employers that they are hiring competent professionals.
Competitive Advantage: Employers can gain a competitive advantage by having a workforce with recognized qualifications and skills.
Reduced Training Costs: Graduates of accredited programs often require less additional training, reducing onboarding costs.
5. Challenges and Criticisms of Accreditation in Data Science Education:
While accreditation offers numerous benefits, it is not without its challenges and criticisms. Some common concerns include:
Bureaucracy: The accreditation process can be lengthy and bureaucratic, causing delays in program development and revisions.
Cost: Preparing for and undergoing accreditation can be expensive, which may result in higher tuition costs for students.
Standardization vs. Innovation: Some argue that accreditation can stifle innovation by promoting conformity over experimentation in education.
Limited Accountability: Accrediting bodies may not always hold institutions accountable for the quality of education they provide, leading to variation in program quality.
Inadequate Standards: Sometimes accrediting standards might not keep up with the fast-evolving discipline of data science, which could lead to curricula that are out of date.
In the ever-changing world of data science education, accreditation plays a crucial role in ensuring high-quality education, industry relevance, and trust from students and employers. While there are challenges and criticisms, such as concerns about bureaucracy, costs, and limitations on innovation, the benefits of accreditation in promoting standardized and excellent education cannot be underestimated.
Accreditation is crucial in maintaining the quality and credibility of data science education. It sets high standards and evaluation processes that not only confirm the program's adherence to industry best practices but also improve students' learning experiences and future opportunities. Although it can be challenging, such as the risk of outdated curricula and limitations on innovation, accreditation helps employers identify competent professionals. It is essential for the continued growth and advancement of data science education by maintaining educational standards and promoting industry relevance.