Power of Learning Communities in Data Science Courses
Discover learning communities, data science, courses, collaboration, skills, networking, support, knowledge sharing, mentorship, growth, and success.
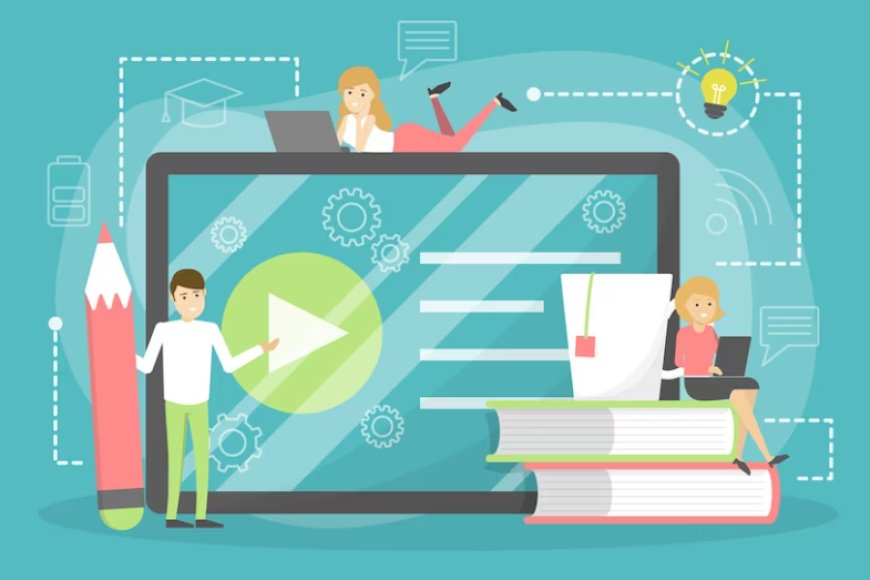
Within the quickly changing field of data science, where the demand for skilled professionals continues to rise, the role of learning communities has emerged as a pivotal force in shaping the educational experiences of students and professionals alike. These communities, characterized by their collaborative and knowledge-sharing ethos, provide a supportive environment for individuals to learn, grow, and thrive in the dynamic field of data science. This article aims to delve into the significance of learning communities within the context of data science courses, highlighting their role in fostering collaboration, facilitating knowledge exchange, and contributing to the overall professional development of participants.
The importance of data science has grown significantly across industries such as finance, healthcare, marketing, and technology. With the increasing reliance on data-driven insights to make informed decisions and stay ahead of the competition, the demand for skilled data scientists has surged. To meet this demand, educational institutions and online platforms have introduced data science courses. However, the constantly evolving nature of data science poses unique challenges for both learners and educators. To address these challenges, learning communities have emerged as an effective strategy to facilitate a more comprehensive learning experience.
The lack of experience with practical applications, traditional classroom settings typically rely on theoretical teaching methods, which may not adequately prepare students for the practical demands of the data science field.
Some specific challenges include:
-
Limited Hands-on Experience: Students may not have the chance to work with real-world datasets or engage in hands-on projects that mirror the challenges faced in the data science industry. This limitation can hinder their ability to apply theoretical knowledge to practical scenarios and develop the problem-solving skills necessary for data science roles.
-
Inadequate Exposure to Industry Tools: Traditional classrooms may lack access to the latest data science tools and technologies commonly used in the industry. Without exposure to these tools, students may find it challenging to adapt to the dynamic technological landscape and may struggle to meet the demands of modern data science jobs.
-
Lack of Industry-Driven Insights: Classroom learning often lacks insights from industry experts and professionals, which can provide valuable context and practical understanding of how data science is applied in real-world settings. Without this industry-driven perspective, students may graduate with a theoretical understanding but may struggle to navigate the complexities of real data science projects and challenges.
-
Limited Collaboration Opportunities: Traditional classroom settings may not foster collaboration among students and with industry professionals, limiting the opportunity for students to learn from diverse perspectives and experiences. Collaboration is essential in the field of data science, as it enables individuals to tackle complex problems collectively and fosters a culture of continuous learning and improvement.
What are Learning Communities in Data Science Courses?
Learning communities within data science courses refer to groups of students and instructors who come together to share knowledge, insights, and experiences related to data science. These communities can exist within traditional classroom settings, online forums, or social media platforms dedicated to data science education. They serve as spaces for collaborative learning, problem-solving, and professional networking, providing students with a supportive environment to enhance their understanding of complex data science concepts.
Benefits of Learning Communities in Data Science Courses
Peer Learning and Collaboration: Learning communities foster peer-to-peer learning, allowing students to exchange ideas, discuss challenging topics, and solve problems collectively. This collaborative approach not only enhances comprehension but also encourages critical thinking and creativity among participants.
Diverse Perspectives and Insights: By bringing together individuals from diverse backgrounds and skill sets, learning communities facilitate the sharing of unique perspectives and insights. This diversity broadens students' understanding of data science applications in various industries and encourages innovative thinking when approaching complex data-related problems.
Mentorship and Guidance: Experienced professionals and educators often participate in these communities, offering mentorship and guidance to students. Their practical knowledge and industry insights provide valuable direction to learners, helping them navigate the intricacies of data science careers and make informed decisions about their professional paths.
Networking Opportunities: Learning communities serve as valuable networking platforms, enabling students to connect with peers, mentors, and industry professionals. These connections can lead to collaborative projects, job opportunities, and the exchange of valuable resources, ultimately enriching the students' learning experience and enhancing their professional growth.
Practical application of skills within learning communities can take various forms, such as:
-
Hands-on Projects: Students can engage in hands-on data science projects, where they apply their knowledge to analyze real datasets and derive meaningful insights. These projects often simulate industry scenarios, enabling students to gain practical experience and develop a deeper understanding of how data science principles are applied in practice.
-
Collaborative Research: Learning communities can facilitate collaborative research initiatives where students work together to address complex data science problems. This collaborative approach not only encourages teamwork and communication but also provides students with the opportunity to contribute to cutting-edge research in the field.
-
Internships and Industry Partnerships: Some learning communities establish partnerships with industry organizations, offering students the chance to participate in internships or work on real projects under the guidance of experienced professionals. This practical exposure allows students to bridge the gap between academic learning and industry demands, equipping them with the necessary skills and experience for successful careers in data science.
-
Case Studies and Simulations: Integrating case studies and simulations into the curriculum allows students to analyze and solve data-related challenges that mimic real-world situations. By engaging in these exercises, students can develop a holistic understanding of how data science concepts are applied in various industries and contexts, preparing them for the complexities they may encounter in their future careers.
-
Workshops and Training Programs: Learning communities often organize workshops and training programs that focus on specific data science tools, technologies, or methodologies.
These hands-on training sessions enable students to gain practical skills that are directly applicable to the data science industry, enhancing their employability and professional competence.
Building and Maintaining Effective Learning Communities in Data Science Courses
To establish and maintain effective learning communities within data science courses, educators and students can take the following steps:
Facilitate Regular Interaction: Encourage regular and meaningful interactions among students through group projects, discussion forums, and collaborative problem-solving activities.
Foster Inclusivity and Diversity: Promote an inclusive environment that values diverse perspectives and encourages active participation from students with varying levels of expertise and backgrounds.
Promote Mentorship and Guidance: Encourage the participation of industry professionals and experienced mentors who can provide guidance and support to students as they navigate the complexities of data science.
Utilize Technology for Collaboration: Leverage technological tools and platforms to facilitate seamless communication, resource sharing, and virtual collaboration among community members, regardless of geographical location.
Encourage Active Learning: Design activities and projects that encourage active learning and require students to apply their knowledge and skills to real-world data science problems.
The importance of learning communities in the ever-changing field of data science education cannot be understated. These communities play a vital role in fostering collaboration, enhancing learning outcomes, and preparing students for the practical challenges of the data science industry. By leveraging the diverse perspectives, mentorship opportunities, and collaborative environments offered by learning communities, students can develop a holistic understanding of data science concepts and establish valuable connections that propel their professional growth in this ever-evolving field. Embracing and nurturing learning communities within data science courses is key to equipping the next generation of data scientists with the skills, knowledge, and network necessary to excel in their careers.