Data Science Bootcamps vs Traditional University Programs
Discover which option suits your learning style and career goals. Make an informed decision for your Data Science education
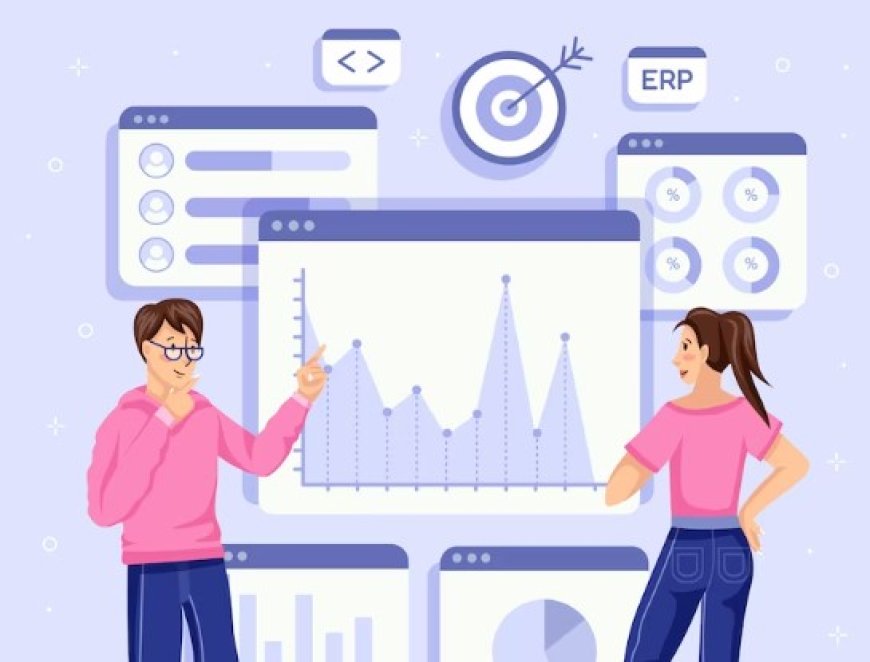
When it comes to choosing between a data science bootcamp and a traditional university program, there are several factors to consider.
Firstly, let's explore the advantages of attending a data science bootcamp. One of the main benefits is the speed at which you can acquire the necessary skills. Bootcamps are typically shorter in duration, ranging from a few weeks to a few months, allowing you to enter the job market quickly. They often have a strong focus on practical skills, providing hands-on experience with practical projects. This can be particularly appealing if you are eager to start working in the field as soon as possible.
Also, bootcamps often have a more industry-oriented curriculum. They are designed to teach you the specific skills and tools that are in demand in the job market. This can give you a competitive edge when applying for data science positions. Bootcamps also tend to have smaller class sizes, allowing for more personalized attention and mentorship from instructors who are experienced professionals in the field.
On the other hand, traditional university programs offer a more comprehensive education. They typically cover a wider range of subjects, including mathematics, statistics, computer science, and domain-specific knowledge. This can provide you with a solid theoretical foundation and a deeper understanding of the underlying principles of data science. University programs also often offer opportunities for research and internships, allowing you to gain valuable experience and make connections in the field.
Still, university programs can be more time-consuming, typically taking four years to complete. This means that it will take longer for you to enter the job market compared to a bootcamp. Additionally, university tuition fees can be quite high, and you may need to take on student loans to finance your education. This can result in a significant financial burden that may take years to repay.
The growth of Data Science Bootcamps
Amidst the limitations of traditional education, data science bootcamps have emerged as a viable alternative. These intensive, short-term programs focus on practical, hands-on training tailored to meet the industry's immediate needs. Typically ranging from a few weeks to a few months, bootcamps prioritize teaching relevant technical skills, tools, and methodologies directly applicable to practical scenarios.
Bootcamps often boost industry-oriented approach, collaborating closely with businesses and professionals to design curriculum content that addresses current market demands. The condensed timeline and concentrated nature of these programs aim to swiftly equip individuals with the skills required to transition into data science roles, making them particularly attractive to career-switchers or those seeking simple entry into the field.
Comparative Analysis
When comparing data science bootcamps and traditional university programs, several key factors come into play:
University Programs:
Depth of Knowledge: University programs in data science or related fields offer a deep dive into theoretical foundations. They cover a wide array of subjects beyond data science itself, including mathematics, statistics, computer science, and domain-specific knowledge. This comprehensive education fosters a holistic understanding, laying a robust groundwork for tackling complex data problems.
Research and Academic Opportunities: The academic environment of universities encourages research initiatives, providing students with opportunities to delve into cutting-edge research projects. This exposure not only contributes to the academic community but also nurtures critical thinking, problem-solving, and innovation. It prepares individuals for roles requiring a deep understanding of theoretical concepts and the ability to contribute to advancing the field through research.
Interdisciplinary Learning: University programs often allow for interdisciplinary learning, enabling students to explore related fields or specialized areas of interest. This breadth of knowledge can be advantageous for those aiming for roles that require a fusion of multiple disciplines, such as bioinformatics, computational finance, or social science research involving data analysis.
Network and Academic Resources: The university setting provides access to diverse academic resources, including libraries, laboratories, and expert faculty members. Moreover, the networks built during university years, both with professors and fellow students, can foster lifelong connections beneficial for future collaborations or career growth.
Data Science Bootcamps:
Industry-Relevant Skills: Bootcamps cater specifically to the skills demanded by the industry. They focus on teaching the latest tools, technologies, and methodologies directly applicable to practical scenarios. This practical emphasis ensures graduates are job-ready with hands-on experience in using industry-standard tools.
Accelerated Learning and Practical Application: The condensed timeline of bootcamps accelerates learning and skill acquisition, allowing individuals to swiftly transition into data science roles. The hands-on approach, often through real projects and case studies, provides practical application experience, bridging the gap between theory and practice.
Industry Collaboration and Job Placement: Bootcamps frequently collaborate with industry partners, providing networking opportunities, guest lectures, and sometimes even job placement assistance. This direct engagement with industry professionals can lead to quicker job placements and a smoother transition into the workforce.
Cost-Effectiveness and Return on Investment: With shorter durations and often lower tuition fees compared to university programs, bootcamps offer a more cost-effective option for gaining relevant skills. The quicker entry into the job market results in a faster return on investment, appealing to individuals seeking immediate career advancement.
Choosing Between Industry Relevance and Academic Depth:
Industry Alignment: Data science bootcamps often maintain close ties with industry professionals and companies. This alignment allows bootcamps to adapt swiftly to changing industry needs, ensuring that the skills taught remain relevant and in demand. This real-time responsiveness to industry trends gives bootcamp graduates an edge in entering the job market with up-to-date skills.
Practical Application: While university programs offer a solid theoretical foundation, they might sometimes lack the practical application of skills. Bootcamps, with their hands-on approach, often simulate practical scenarios, providing students with practical experience in solving industry-specific problems. This practical exposure can be invaluable when transitioning into actual data science roles.
Flexibility and Agility: The flexibility of bootcamps in adapting their curriculum allows for the inclusion of the latest tools, techniques, and technologies swiftly. In contrast, the structured nature of university programs might take longer to update course material to reflect industry advancements, potentially causing a lag in teaching emerging skills.
Diversity in Learning Formats: Traditional university programs often follow a standardized format, primarily classroom-based with scheduled lectures and seminars. In contrast, bootcamps might adopt diverse teaching formats, including workshops, online modules, and mentorship programs, catering to various learning styles and preferences.
Job Placement and Alumni Networks: Bootcamps frequently emphasize job placement services and foster strong alumni networks, aiding graduates in securing employment opportunities. These networks and connections within the industry can significantly impact the ease and speed of transitioning into data science roles.
Depth vs. Breadth of Knowledge: University programs tend to provide a deeper understanding of foundational concepts and theories, which can be advantageous for individuals aspiring to pursue advanced research or academia within data science. Conversely, bootcamps prioritize breadth, focusing on practical skills and immediate applicability, ideal for those aiming to swiftly enter the job market.
Continual Learning and Growth: Both pathways encourage continual learning, but their approaches differ. University programs instill a mindset of academic exploration and research, fostering lifelong learning habits. Bootcamps, with their intense and focused nature, might ignite a thirst for ongoing skill enhancement to keep up with the changing data science environment.
Both data science bootcamps and traditional university programs have their own advantages and disadvantages. If you're looking for a quick and intensive learning experience with a focus on practical skills, a bootcamp might be the
In the end, the choice between a data science bootcamp and a traditional university program depends on individual preferences, career goals, financial considerations, and preferred learning styles. While bootcamps cater to a more accelerated and practical approach, university programs offer a more holistic and theoretical foundation. As the field of data science continues to evolve, both educational paths will play essential roles in shaping the next generation of data scientists, catering to the diverse needs of learners aspiring to thrive in this dynamic field.
Refer these articles:
- Data Visualization: Techniques and Tools for Visualizing Data
- Data Science Course Specializations
- Effective Teaching Methods for Data Science Courses: A Comprehensive Guide