Learning Outcomes of a Data Science Course
Understand analytics, modeling, algorithms, statistics, machine learning, AI, visualization, big data, Python, and insights. Master data science skills.
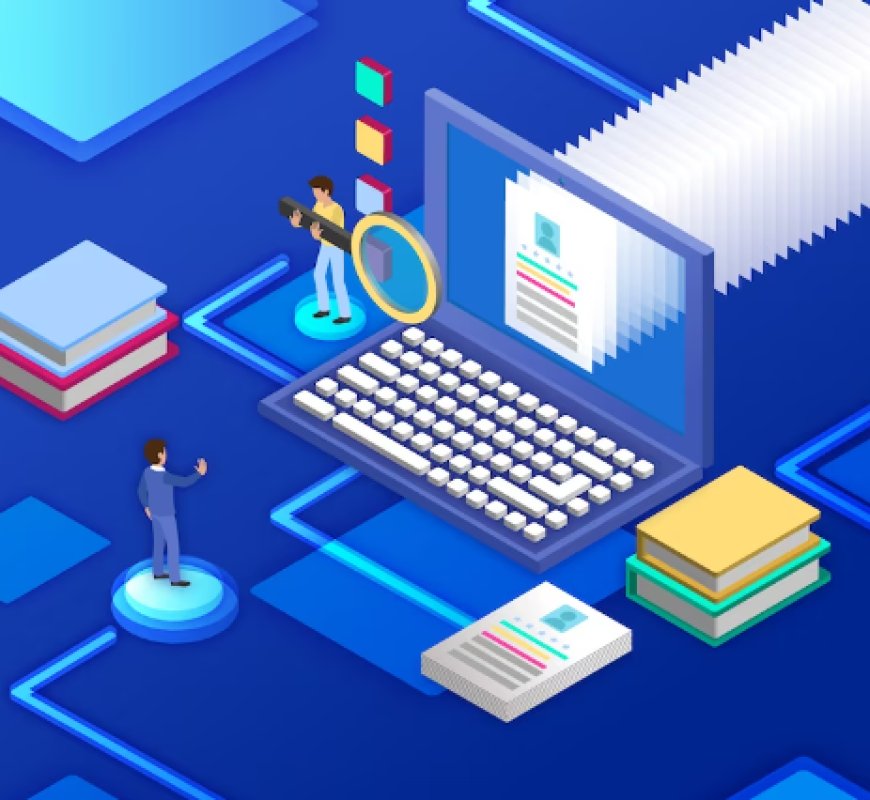
Data science has emerged as a transformative field that leverages the power of data to extract valuable insights, drive innovation, and make informed decisions. As the demand for skilled data scientists continues to rise, data science courses have become a popular choice for students aspiring to enter this dynamic field. In this blog, we will delve into the essential learning outcomes of data science courses and explore how they equip students with the knowledge and skills necessary to thrive in the world of data.
Proficiency in Data Manipulation and Analysis
One of the key learning outcomes of data science courses is the development of proficiency in data manipulation and analysis. In the world of data science, working with large and complex datasets is a common occurrence. Students are equipped with the necessary skills to handle data effectively and derive valuable insights from it.
Data manipulation involves tasks such as cleaning, preprocessing, and transforming raw data into a format suitable for analysis. Students learn techniques to handle missing data, handle outliers, and perform data imputation. They gain proficiency in using programming languages like Python or R, as well as data manipulation libraries such as Pandas, to efficiently manipulate and organize data.
Data analysis is another crucial aspect of data science courses. Students learn a variety of statistical methods and techniques to explore and analyze data. They acquire knowledge of descriptive statistics to summarize and understand the characteristics of datasets. Students also delve into inferential statistics, hypothesis testing, and confidence intervals to make inferences and draw conclusions about the population from sample data.
Students also learn how to apply various data analysis techniques, such as regression analysis, time series analysis, and clustering. Regression analysis enables them to model relationships between variables and make predictions. Time series analysis equips them with the ability to analyze data with a temporal component, such as stock prices or weather patterns. Clustering techniques allow students to group similar data points together, aiding in segmentation and pattern discovery.
Proficiency in data manipulation and analysis is essential for data scientists to effectively extract valuable insights and make informed decisions. It enables them to handle diverse and messy real-world datasets and uncover meaningful patterns and relationships. By mastering these skills, students are well-prepared to tackle the challenges of working with data and leverage its potential for driving innovation and solving complex problems.
Understanding Statistical Concepts and Modeling Techniques
Data science courses emphasize the importance of statistical concepts and modeling techniques. Students learn statistical methods to make sense of data, understand its distribution, and uncover patterns and relationships. They explore various modeling techniques, including regression analysis, classification, clustering, and time series analysis, to build predictive and descriptive models. Understanding these concepts enables students to make data-driven decisions, develop accurate predictions, and identify actionable insights.
Proficiency in Machine Learning and Artificial Intelligence
Machine learning and artificial intelligence (AI) play a vital role in data science. Data science courses equip students with the knowledge and skills to apply machine learning algorithms and AI techniques to analyze and interpret data. Students learn to train models, evaluate their performance, and apply them to solve real-world problems such as image recognition, natural language processing, recommender systems, and anomaly detection. Proficiency in machine learning and AI empowers students to leverage advanced techniques and technologies for data-driven solutions.
Data Visualization and Communication
Data science courses emphasize the importance of effective data visualization and communication skills. Students learn to create visually appealing and informative data visualizations to convey insights and tell compelling data stories. They gain proficiency in using visualization tools and techniques to present complex data in a comprehensible manner. Additionally, students learn to communicate their findings to both technical and non-technical audiences, enabling them to effectively communicate the value and impact of data-driven insights.
Ethical and Responsible Data Science
Data science courses instill a strong ethical foundation in students. They learn about the ethical implications of working with data, including privacy concerns, bias, and fairness. Students understand the importance of responsible data collection, handling, and analysis to ensure the ethical use of data. They develop critical thinking skills to evaluate the ethical implications of data science practices and make informed decisions that prioritize transparency, accountability, and privacy protection.
Collaborative and Problem-Solving Skills:
In addition to technical knowledge, data science courses emphasize the development of collaborative and problem-solving skills. Data science projects often require interdisciplinary collaboration and a team-based approach to tackle complex problems. Students are encouraged to work together, share ideas, and leverage diverse perspectives to find innovative solutions.
Collaboration is a vital skill in data science as it simulates real-world scenarios where data scientists work alongside domain experts, software engineers, and business stakeholders. Data science courses create opportunities for students to engage in group projects and case studies, where they learn to collaborate effectively. They gain experience in communicating and coordinating with team members, dividing tasks, and leveraging individual strengths to achieve common goals.
Through collaborative projects, students also learn the importance of active listening, constructive feedback, and conflict resolution. They develop the ability to navigate different opinions, negotiate compromises, and foster a positive team dynamic. These skills are crucial for successful teamwork and ensuring that diverse perspectives are valued and integrated into the problem-solving process.
Problem-solving skills are essential in data science, where complex and ambiguous problems are the norm. Data science courses teach students various problem-solving techniques and frameworks, such as the CRISP-DM (Cross-Industry Standard Process for Data Mining) methodology. Students learn to define problem statements, identify data requirements, formulate hypotheses, and design experiments or models to test their hypotheses.
Moreover, students gain experience in identifying and addressing data-related challenges, such as data quality issues, bias, or missing information. They develop critical thinking skills to evaluate different approaches and select the most suitable methods for data analysis and modeling. This analytical mindset enables them to break down complex problems into manageable components and systematically analyze and solve them.
Collaborative problem-solving also extends beyond technical aspects. Students are encouraged to consider ethical implications, social impact, and business constraints when designing solutions. They learn to strike a balance between technical feasibility and ethical responsibility, ensuring that their solutions align with ethical standards and regulatory requirements.
By developing collaborative and problem-solving skills, data science students become well-rounded professionals capable of working effectively in interdisciplinary teams and addressing real-world challenges. These skills enhance their ability to communicate complex ideas, adapt to diverse working environments, and approach problem-solving from multiple perspectives.
Data science courses offer a comprehensive set of learning outcomes that equip students with the knowledge and skills necessary to excel in the field of data science. From data manipulation and analysis to statistical modeling, machine learning, and data visualization, students gain a holistic understanding of data science techniques and tools. Moreover, they develop critical thinking, communication, and ethical decision-making skills that are essential for responsible and impactful data science practices. By achieving these learning outcomes, students are well