Ethics of Data Science: A Friendly Guide
Explore data science ethics, principles, privacy, AI, transparency, accountability, biases, practices, integrity, challenges, regulations.
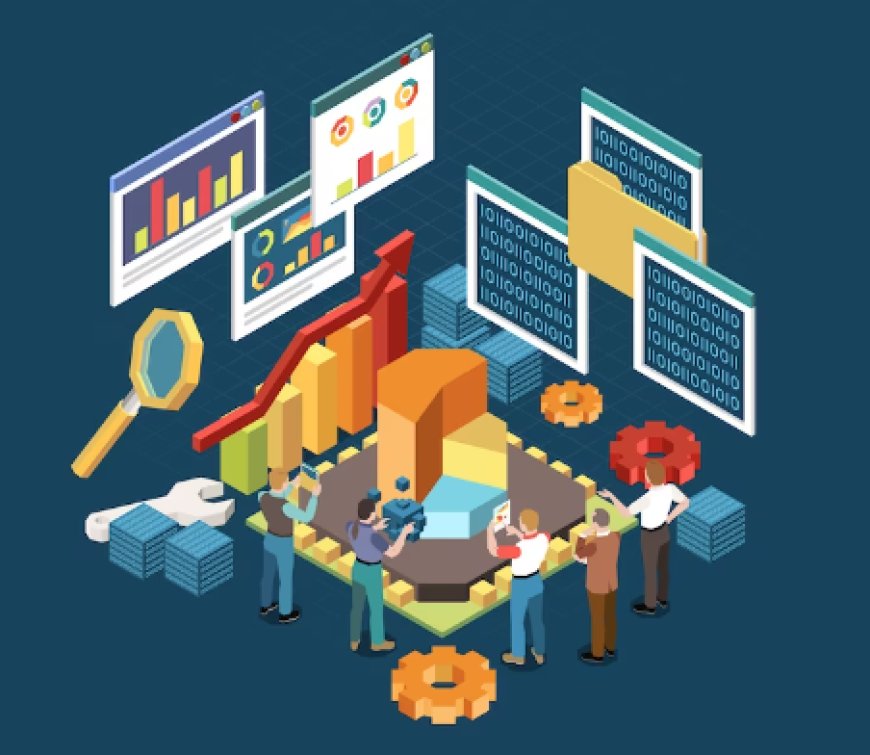
Welcome, dear readers, to our friendly guide on the captivating world of data science ethics! In this digital age, where data is abundant and algorithms rule the realm, it's crucial for us to explore the ethical implications that accompany this fascinating field. So, grab a cup of coffee, sit back, and let's embark on this journey together.
The Rise of Data Science
Data science has become a formidable force in today's society. Its vast potential has been harnessed across various domains, from healthcare to finance, education to marketing. Thanks to the abundance of data, scientists can unveil valuable insights, predict outcomes, and make informed decisions. However, with great power comes great responsibility.
The Ethical Imperative
As data scientists, we possess the power to shape our society and influence the lives of individuals. It is our ethical duty to ensure that our work upholds integrity, fairness, and respect for privacy. Let's dive into a few key ethical considerations that should guide us on this path.
Data Privacy and Consent
Data privacy refers to the protection of individuals' personal information, ensuring that it is collected, stored, and used in a secure and confidential manner. In the digital age, where vast amounts of data are generated and shared, maintaining privacy has become increasingly challenging. That's why it is crucial for data scientists to implement robust security measures, encryption protocols, and access controls to safeguard sensitive data.
However, data privacy goes beyond mere technical safeguards. It involves respecting the boundaries and expectations individuals have regarding their personal information. This is where consent plays a pivotal role. Consent is the explicit permission granted by individuals for their data to be collected, processed, and shared for specific purposes.
Obtaining informed consent is a fundamental principle in data science ethics. It means being transparent about how data will be used, who will have access to it, and for what purposes. Consent should be freely given, without coercion or deceptive practices. Individuals should have the right to understand and control how their data is being utilized, and they should be able to revoke their consent at any time.
As data scientists, it is our responsibility to ensure that individuals are well-informed about the implications of sharing their data. This means clearly communicating the benefits, risks, and potential consequences associated with data collection and processing. It also involves providing individuals with options to exercise their rights, such as the ability to opt-out, request data deletion, or rectify inaccuracies.
Respecting data privacy and obtaining informed consent not only aligns with ethical principles, but it also builds trust between organizations and individuals. When individuals trust that their personal information will be handled responsibly, they are more likely to engage with data-driven initiatives and contribute their data for research or analysis. Trust is the foundation upon which data science can thrive and deliver meaningful insights that benefit society.
Fairness and Bias
Algorithms hold the potential to perpetuate societal biases if not carefully developed and deployed. It's essential to critically examine the data we use, as biased datasets can lead to biased results. Striving for fairness means actively seeking diversity and representation, questioning our assumptions, and continuously evaluating and mitigating bias throughout the entire data science pipeline.
Responsible AI Development
Responsible AI development is crucial for ensuring that artificial intelligence technologies are designed and deployed in a manner that aligns with ethical and societal considerations. It encompasses a set of principles and practices aimed at mitigating potential risks and maximizing the positive impact of AI systems.
First and foremost, responsible AI development emphasizes transparency and explainability. Developers should strive to create AI models and algorithms that are understandable and interpretable, enabling users and stakeholders to comprehend how decisions are made. This helps build trust and allows for accountability in AI systems, ensuring that outcomes can be justified and verified.
Equity and fairness are also fundamental aspects of responsible AI development. Developers must be mindful of potential biases in data collection and algorithmic decision-making processes. They should work to mitigate biases and ensure that AI systems do not perpetuate or amplify discrimination or inequality. Attention should be given to diverse representation in training data and continuous monitoring to address biases that may emerge during deployment.
Responsible AI development also includes considerations for privacy and data protection. Developers should prioritize the security and confidentiality of user data, adhering to legal and ethical standards. Clear policies and procedures must be in place to govern data collection, storage, and usage, and individuals' rights to consent and control over their data must be respected.
Additionally, responsible AI development promotes ongoing monitoring and evaluation of AI systems. Regular assessments should be conducted to identify potential risks and unintended consequences, with mechanisms in place to address and rectify any issues that arise. Continuous learning and improvement are essential to ensure that AI technologies evolve in a responsible and beneficial manner.
Collaboration and engagement with stakeholders, including the public, are crucial components of responsible AI development. Developers should actively seek input, feedback, and diverse perspectives to inform the design and deployment of AI systems. Engaging in open dialogue and soliciting input helps to ensure that AI technologies are aligned with societal values and needs.
Data Security and Cybersecurity
Data Security and Cybersecurity: Safeguarding the Digital Frontier
In today's interconnected world, where data is the lifeblood of organizations and technology permeates every aspect of our lives, data security and cybersecurity have become vital concerns. As data scientists, it is imperative for us to prioritize the protection of sensitive information and defend against cyber threats to maintain trust, integrity, and the confidentiality of data.
Data security involves implementing measures to prevent unauthorized access, alteration, or destruction of data. It encompasses a range of practices, including encryption, access controls, secure storage, and regular backups. By implementing these measures, we can ensure that data remains confidential and protected from both external threats and internal vulnerabilities.
Cybersecurity, on the other hand, focuses on safeguarding digital systems and networks from malicious activities. It involves identifying and mitigating vulnerabilities, detecting and responding to attacks, and continuously monitoring for potential threats. Cybersecurity measures include firewalls, intrusion detection systems, antivirus software, and employee awareness training. A strong cybersecurity strategy is crucial in preventing data breaches, unauthorized data access, and other malicious activities that can compromise the security and integrity of data.
As data scientists, we play a vital role in data security and cybersecurity. We must be vigilant in safeguarding data throughout its lifecycle, from collection to analysis and storage. This means implementing best practices in data management, securely transferring and storing data, and using encryption techniques to protect sensitive information.
Collaboration is also key in ensuring data security and cybersecurity. It is important to work closely with IT departments, security experts, and stakeholders to establish robust security protocols, conduct risk assessments, and implement necessary security controls. By fostering a culture of security awareness and promoting good cybersecurity practices within organizations, we can create a strong defense against cyber threats.
Social and Environmental Impact
Data science has the potential to address pressing social and environmental challenges. We should actively consider the broader impact of our work, striving to contribute positively to society and the planet. Whether it's using data to improve healthcare access or creating models for sustainable resource management, data scientists can be a driving force for positive change.
As data scientists, we possess the keys to unlock the vast potential of data. However, we must wield this power responsibly and ethically. By prioritizing privacy, fairness, transparency, and societal impact, we can ensure that data science remains a force for good.
Together, we can shape a world where data science empowers and benefits all while upholding the values we hold dear. Happy data science exploration, and remember: ethics will always be our North Star