The Role of Internships and Projects in Kickstarting Your Data Science Career
Discover how practical experiences contribute to skill development, industry exposure, and career advancement in the dynamic field of data science
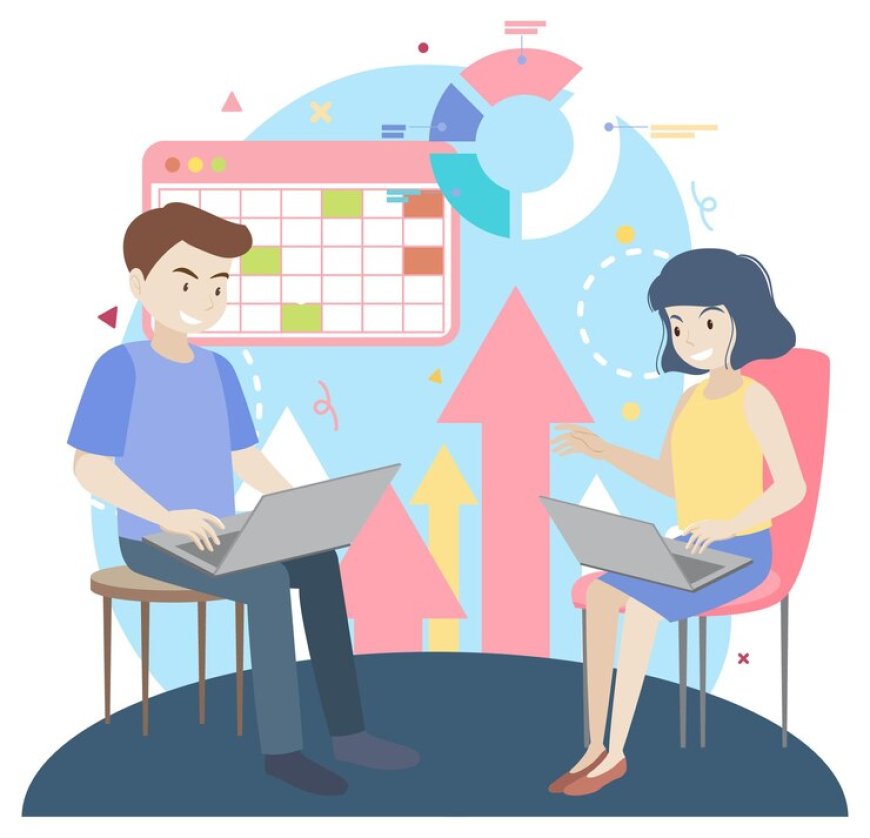
Data science internships and projects refer to practical learning opportunities where individuals apply their theoretical knowledge of data science in real-world scenarios. These experiences often involve working on data-centric tasks, analyzing large datasets, implementing machine learning models, and solving complex business challenges using data-driven insights. Data science internships and projects aim to provide hands-on experience and exposure to the practical applications of data science principles and techniques in various industries and domains.
Practical experience plays a crucial role in the launch of a data science career as it enables individuals to apply their theoretical knowledge in real-world contexts, gain exposure to industry practices, and develop essential skills required for data-driven roles. Engaging in data science internships and projects allows individuals to hone their analytical, problem-solving, and critical thinking abilities, while also fostering a deeper understanding of data analysis, interpretation, and communication. Practical experience not only enhances a candidate's employability but also demonstrates their proficiency and readiness to tackle complex data-centric challenges in the professional sphere.
Importance of Internships in Data Science
-
Hands-on experience with real-world data science applications and tools
-
Exposure to industry practices and workflows within the data science domain
-
Opportunities to collaborate with professionals and experts in the field
-
Development of practical skills in data analysis, modeling, and problem-solving
-
Application of theoretical knowledge to real-world data science projects and challenges
-
Enhancement of critical thinking and decision-making abilities in data-driven contexts
-
Networking opportunities and connections with industry professionals and mentors
-
Building a strong foundation for a successful career in the competitive field of data science
-
Demonstrating practical experience and industry readiness to prospective employers
-
Gaining insights into the day-to-day responsibilities and expectations of a data science role
Types of Data Science Projects
Independent research projects and case studies in data science involve individual exploration and analysis of specific research questions or datasets. These projects allow aspiring data scientists to delve into self-directed inquiries, apply various data science methodologies, and draw meaningful conclusions. Through independent research, individuals can showcase their problem-solving skills, research acumen, and the ability to derive actionable insights from complex data sets, highlighting their capacity for independent work and critical analysis.
Collaborative data science projects with industry partners or academic institutions involve working with external stakeholders to address real-world challenges or research questions. These projects provide opportunities for aspiring data scientists to engage in team-based collaborations, leverage diverse perspectives and expertise, and contribute to solving complex problems that have practical implications. Collaborative projects foster interpersonal skills, teamwork, and effective communication, while also enabling individuals to build a professional network and gain insights into industry best practices and expectations.
Open-source contributions and community-driven initiatives in data science involve actively participating in public projects, contributing to data science libraries, and collaborating with the broader data science community. By contributing to open-source projects, individuals can demonstrate their coding proficiency, problem-solving abilities, and commitment to knowledge sharing and collaboration within the data science field. Open-source contributions also provide valuable opportunities for individuals to receive feedback, engage in peer learning, and establish their presence within the larger data science community, showcasing their dedication to continuous learning and professional growth.
Benefits of Data Science Projects
Data science internships and projects provide an opportunity for individuals to develop and refine their skills in utilizing various data tools and technologies. Through practical experience, interns and project participants gain proficiency in using programming languages, statistical software, data visualization tools, and other data science-related technologies, enhancing their technical capabilities and familiarity with industry-standard tools.
Engaging in data science internships and projects allows individuals to build a robust portfolio that highlights their practical expertise and accomplishments in data analysis, modeling, and problem-solving. A well-curated portfolio featuring successful project outcomes, data-driven insights, and impactful contributions demonstrates the candidate's hands-on experience and showcases their ability to apply theoretical knowledge to real-world scenarios, making them more attractive to potential employers.
Data science internships and projects offer a platform for individuals to demonstrate their problem-solving abilities and critical thinking skills in the context of complex data-centric challenges. By actively participating in data-driven tasks, interns and project participants develop the capacity to approach problems analytically, make data-informed decisions, and derive meaningful insights from complex datasets, showcasing their ability to tackle real-world business problems using a data-driven and evidence-based approach.
Strategies for Securing Data Science Internships
A. Leveraging networking and professional connections: Networking and professional connections can significantly enhance your chances of securing a data science internship. Engage with professionals in the field through industry events, online forums, and social media platforms to gain insights, advice, and potential referrals for internship opportunities. Building strong relationships within the data science community can provide valuable mentorship, guidance, and access to exclusive internship openings.
B. Crafting a compelling resume and cover letter tailored to data science roles: A well-crafted resume and cover letter tailored to data science roles are essential for making a positive impression on potential employers. Highlight your relevant skills, academic achievements, and any data science-related projects or experiences. Emphasize your proficiency in data analysis, programming languages, and machine learning techniques, showcasing your ability to contribute to data-driven projects effectively. Tailor your application materials to the specific requirements and expectations of the data science internship you are applying for.
C. Preparing for internship interviews and assessments: Preparation is key to excelling in data science internship interviews and assessments. Familiarize yourself with common data science interview questions, technical assessments, and case studies. Practice your problem-solving and analytical skills, and be prepared to demonstrate your knowledge of data science concepts, methodologies, and tools. Showcase your ability to communicate effectively and articulate your thought process during the interview process. Conduct mock interviews, participate in coding challenges, and seek feedback to refine your interview and assessment performance.
Making the Most of Data Science Internships and Projects
Making the most of data science internships and projects involves actively engaging in practical learning experiences, setting clear objectives for personal and professional growth, seeking mentorship and guidance from industry experts, and documenting achievements and lessons learned for future reference. By leveraging these opportunities, individuals can develop essential skills, build a strong portfolio, and position themselves for a successful career in data science. It is essential to actively participate in hands-on tasks, collaborate with professionals, and apply theoretical knowledge to real-world scenarios, ensuring a comprehensive and impactful learning experience.
Transitioning from Internships and Projects to a Full-fledged Data Science Career
A. Showcasing internship or project experiences in job applications: Highlighting the experiences gained from data science internships and projects in job applications is crucial for demonstrating practical expertise and relevance to potential employers. Showcase the specific tasks, challenges, and outcomes of your internship or project work, emphasizing your contributions, achievements, and the skills developed during the practical experience. Use tangible results, data-driven insights, and successful project outcomes to showcase your capabilities and differentiate yourself as a competitive candidate in the data science job market.
B. Leveraging networking and references for career opportunities: Networking and leveraging professional references play a pivotal role in accessing career opportunities and securing data science roles. Cultivate and maintain strong professional relationships with industry peers, mentors, and former colleagues to expand your professional network. Leverage these connections for insights into job openings, industry trends, and potential career opportunities. Seek recommendations and endorsements from professionals who can attest to your skills, work ethic, and contributions, enhancing your credibility and visibility in the competitive data science job market.
C. Continuous learning and skill development for career advancement: Continuous learning and skill development are essential for staying competitive and advancing in a data science career. Engage in ongoing education, training programs, and professional development opportunities to stay updated with the latest trends, tools, and techniques in data science. Pursue certifications, attend workshops, and participate in industry conferences to broaden your knowledge and expertise. Cultivate a growth mindset, embrace new challenges, and actively seek opportunities to expand your skill set and stay ahead of the evolving demands and advancements in the dynamic field of data science.
Engaging in practical experiences allows individuals to refine their problem-solving abilities, enhance their analytical skills, and develop a deeper understanding of data analysis techniques. These experiences also provide opportunities to collaborate with professionals in the field, learn from their expertise, and build a strong professional network. By actively seeking practical experiences, aspiring data scientists can demonstrate their commitment to personal and professional growth, positioning themselves as competent and competitive candidates in the dynamic and rapidly evolving field of data science.