Effective Teaching Methods for Data Science Courses: A Comprehensive Guide
Discover effective teaching methods for data science courses in this comprehensive guide. Explore innovative strategies and techniques to enhance data science education
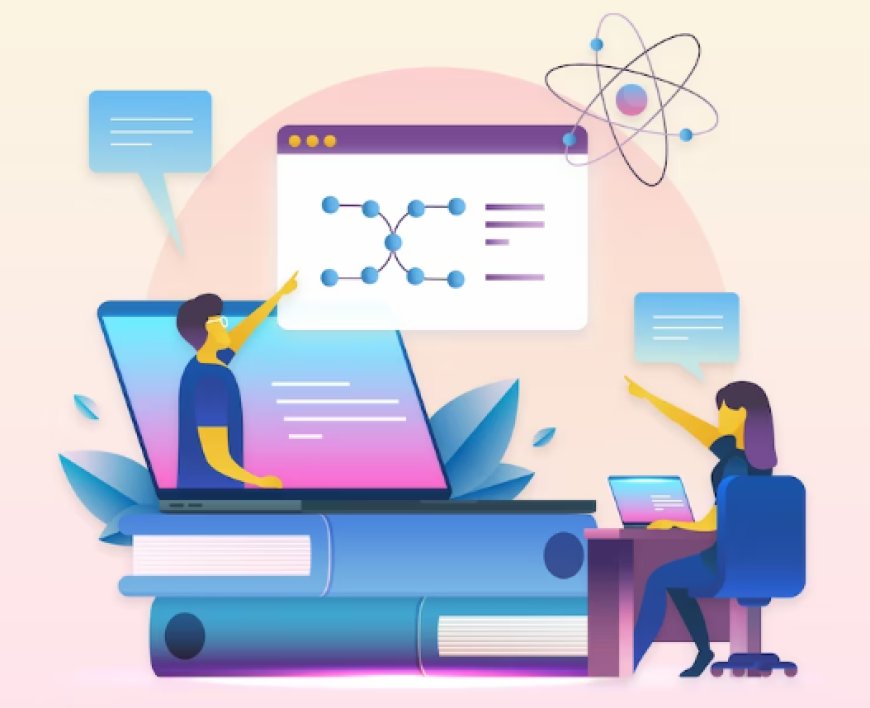
Data science education is becoming more and more important in preparing students for the modern workforce. It provides them with the necessary skills to analyze and make sense of complex data sets. As data continues to grow in different fields, such as business and healthcare, the ability to understand and utilize data has become highly valued. Effective teaching methods play a crucial role in helping students grasp and excel in data science concepts. These methods involve creative strategies and techniques that actively engage students, encourage critical thinking, and foster a strong comprehension of data science principles and their practical applications.
The state of data science education currently reveals a growing emphasis on practical applications, Including practical projects and case studies to provide hands-on experience. Many institutions offer diverse specialized courses catering to various industries, including healthcare, finance, and technology. Additionally, there is a shift towards integrating interdisciplinary subjects, such as machine learning and artificial intelligence, to equip students with a comprehensive skill set.
Students and educators encounter various challenges in data science, including the complexity of the subject matter, the quick advancement of technology, and the demand for updated curricula and resources. Educators face the challenge of staying current with the latest advancements while creating engaging and practical learning experiences. Students often struggle with the technical intricacies and the interdisciplinary nature of data science, requiring comprehensive support and guidance to navigate the complexities of the field.
Strategies for Successful Data Science Instruction
Teaching data science effectively involves addressing a range of challenges, including the integration of diverse case studies to indicate practical applications. This process also entails incorporating various computing skills and statistical concepts, balancing theoretical knowledge with practical implementation, and keeping up with the dynamic nature of the field. Finally, instructors need to foster a comprehensive understanding of data science by emphasizing critical thinking, problem-solving, and interdisciplinary collaboration among students.
-
Rapid Technological Advancements: The rapid evolution of data science technologies and tools poses a challenge for instructors to keep pace with the latest advancements and integrate them into the curriculum effectively. This necessitates continuous professional development and a proactive approach to staying updated with the latest industry trends and best practices.
-
Diverse Student Backgrounds: Students enrolling in data science courses often come from diverse educational backgrounds, with varying levels of familiarity with mathematical concepts, programming languages, and data analysis techniques. Addressing the diverse needs and skill levels of students while maintaining an engaging and cohesive learning experience can be a significant challenge for instructors.
-
Scalability of Practical Training: Providing hands-on training and practical experience in data science often requires access to substantial computing resources, datasets, and specialized software, which might not be easily accessible to all educational institutions. Ensuring the scalability and accessibility of practical training can pose logistical and resource-related challenges for instructors and educational institutions.
-
Interdisciplinary Nature of Data Science: Data science intersects with various disciplines, including computer science, mathematics, and domain-specific knowledge areas. Effectively integrating interdisciplinary concepts and fostering cross-disciplinary collaboration within the educational framework demands a comprehensive understanding of multiple domains, which can be challenging for instructors coming from specialized backgrounds.
-
Dynamic Curriculum Development: The dynamic nature of the data science field necessitates a curriculum that is adaptable and responsive to the changing demands of the industry. Instructors face the challenge of continually updating and refining the curriculum to include emerging technologies, methodologies, and best practices, ensuring that students are equipped with the most relevant and up-to-date skills upon graduation.
-
Complexity of Big Data Analysis: Teaching the intricacies of handling and analyzing large-scale datasets, commonly referred to as big data, presents a significant challenge. Educators need to emphasize scalable data processing techniques, distributed computing, and data visualization methods to equip students with the necessary skills to address the challenges associated with big data analysis.
Given the intricacies of data science and the diverse needs of learners, what are the most effective teaching methods that instructors can employ to facilitate an enriching and practical learning environment in data science courses?
1. Hands-on Projects and Case Studies:
Integrating hands-on projects and actual case studies into the curriculum allows students to apply theoretical knowledge to practical scenarios. By working on relevant projects, students gain valuable experience in data collection, analysis, and interpretation, thereby strengthening their problem-solving and critical-thinking skills.
2. Interactive Workshops and Seminars:
Organizing interactive workshops and seminars featuring industry experts and experienced professionals provides students with insights into the practical applications of data science in various domains. These sessions encourage active participation and foster a deeper understanding of the practical implications of data science techniques and methodologies.
3. Collaborative Learning and Team Projects:
Encouraging collaborative learning through group projects fosters a cooperative and supportive learning environment. Students can leverage each other's strengths and expertise, leading to the development of teamwork and communication skills, which are essential for success in the field of data science.
4. Blended Learning Approach:
Implementing a blended learning approach that combines traditional classroom instruction with online resources and virtual learning platforms can enhance the overall learning experience. Providing access to interactive online tutorials, virtual labs, and multimedia resources facilitates self-paced learning and accommodates diverse learning styles.
5. Regular Feedback and Assessment:
Offering regular feedback and assessments throughout the course enables instructors to track students' progress and identify areas that require additional focus. Constructive feedback not only encourages continuous improvement but also helps students gauge their strengths and weaknesses, fostering a sense of accountability and self-awareness.
6. Stay Updated with Industry Trends:
To ensure the relevance and currency of the curriculum, instructors must stay abreast of the latest industry trends and technological advancements in the field of data science. Incorporating cutting-edge tools and techniques into the teaching approach can equip students with the skills and knowledge needed to tackle practical data difficulties, keeping the curriculum aligned with industry demands.
7. Personalized Learning Paths:
Recognizing the diverse backgrounds and skill levels of students, and offering personalized learning paths tailored to individual learning needs can significantly enhance the learning experience. Providing supplementary resources, additional support, and advanced modules for students with varying levels of expertise fosters a more inclusive and accommodating learning environment.
8. Practical Internship Opportunities:
Facilitating practical internship opportunities with industry-leading organizations enables students to gain hands-on experience and exposure to actual data science projects in a practical setting. Internships not only supplement theoretical knowledge but also provide invaluable networking opportunities and industry connections, fostering professional growth and career development.
9. Ethical and Legal Implications Awareness:
Emphasizing the ethical and legal implications of data science practices is vital in cultivating responsible and conscientious data professionals. Integrating discussions on data privacy, security, and ethical considerations into the curriculum instills a sense of ethical responsibility and encourages students to approach data science applications with integrity and respect for user privacy.
10. Continuous Learning and Professional Development:
Encouraging a culture of continuous learning and professional development through participation in workshops, conferences, and online courses empowers students to stay updated with the latest advancements in the field. Promoting lifelong learning instills a sense of adaptability and resilience, enabling students to navigate The constantly evolving field of data science with confidence and competence.
Effective teaching methods are crucial in shaping the next generation of data professionals in the constantly shifting field of data science. Educators can create engaging and comprehensive learning experiences by incorporating hands-on projects, interactive workshops, blended learning approaches, and personalized learning paths. It is also important to foster an understanding of ethical and legal implications and promote a culture of continuous learning and professional development to nurture responsible and adaptable data science professionals. By implementing these methods, instructors can cultivate a thriving learning environment that equips students with the necessary skills and knowledge to excel in the ever-evolving field of data science.