Magic of Exploratory Data Analysis: A Journey of Discovery
Uncover hidden insights and patterns, shaping a deeper understanding of your data universe
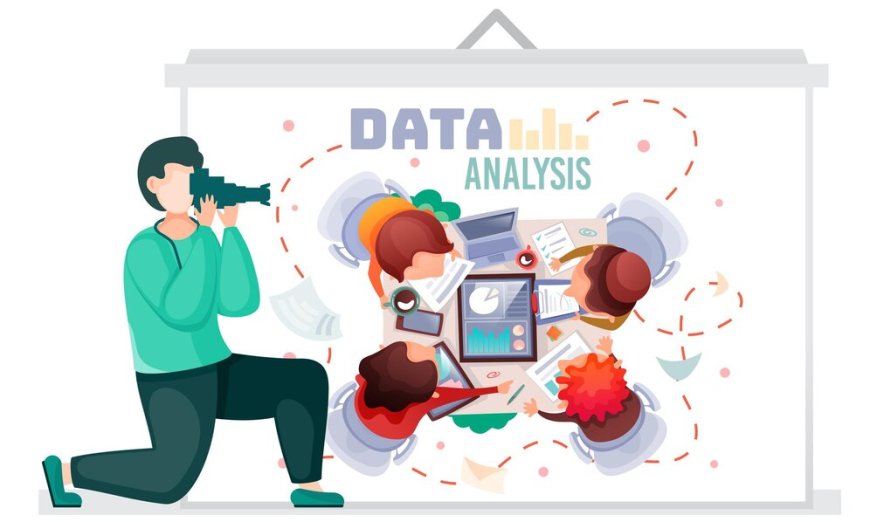
Data is like a hidden treasure that is waiting to be discovered. It has the ability to show valuable insights, patterns, and stories that would otherwise remain unknown. By using Exploratory Data Analysis (EDA), We have the ability to reveal these valuable possessions and gain a better understanding of the underlying patterns and structures within a dataset. With this powerful analytical technique.
In the age of information, we are inundated with data from various sources - be it business transactions, social media interactions, scientific experiments, or IoT sensors. This abundance of data is often referred to as "big data." While big data holds tremendous potential, it also presents significant challenges. One of the major challenges is making sense of the data. That's where EDA comes into play.
The Complexity of Raw Data
Raw data is often messy and complex. It can contain missing values, outliers, inconsistencies, and errors. Without proper data exploration, trying to perform any meaningful analysis is akin to searching for a needle in a haystack. Moreover, data can take various forms - structured, semi-structured, or unstructured - making it even more challenging to extract valuable insights. EDA is absolutely essential because it equips us with the necessary techniques and tools to navigate through this intricate surface.
What Does EDA Involve?
Before diving into the answers, let's clarify what EDA entails. Exploratory Data Analysis is not a singular process, but rather a collection of techniques and methods used to summarize, visualize, and understand data.
Some key components of EDA include:
Descriptive Statistics: These statistics provide an initial overview of the data, including measures like mean, median, and standard deviation. They offer insights into the central tendencies and variability of the data.
Data Visualization: EDA heavily relies on data visualization techniques such as histograms, scatter plots, box plots, and heat maps. These visualizations help in identifying patterns and trends within the data.
Data Cleaning: This step involves handling missing values, outliers, and inconsistencies. It's crucial to ensure that the data is in a suitable condition for analysis.
Data Transformation: EDA may require data transformation, which can involve operations like log transformations, scaling, or encoding categorical variables.
Correlation Analysis: EDA helps in identifying the relationships between variables. It can reveal if one variable is influenced by another or if they are independent.
Pattern Recognition: Through EDA, you can identify patterns, clusters, and trends within the data. This is especially useful in unsupervised machine-learning tasks like clustering.
Anomaly Detection: EDA can uncover anomalies or outliers that may be indicative of errors or unusual events in the data.
Hypothesis Generation: EDA can spark the formulation of hypotheses, which can be tested rigorously in subsequent phases of analysis.
The magic of Exploratory Data Analysis in various aspects:
Data Understanding: EDA offers a comprehensive understanding of the dataset. It provides a glimpse into the data's distribution, central tendencies, and the presence of any extreme values. This initial grasp of the data is crucial for deciding the next steps in the analysis.
Feature Selection: In machine learning and predictive modeling, not all features (variables) are created equal. EDA can help identify which features are most relevant, reducing dimensionality and improving model performance.
Outlier Detection: Outliers, which are data points significantly different from the rest of the dataset, can have a substantial impact on analysis and modeling. EDA helps in identifying and dealing with outliers effectively.
Data Imputation: Missing data is a common issue in real-world datasets. EDA can guide the process of imputing missing values using appropriate techniques, ensuring that the analysis is not compromised.
Visualization: Data visualization is a powerful tool for EDA. Visual representations make complex data more understandable, and patterns that may not be evident in raw numbers can become apparent in a well-constructed chart or graph.
Decision Support: EDA assists in making informed decisions about which statistical methods or machine learning algorithms are suitable for the dataset. It informs the choice of data preprocessing and modeling techniques.
Identifying Data Quality Issues: EDA helps in spotting data quality issues, which could be due to data entry errors, inconsistencies in data collection, or issues with data integration.
Domain Knowledge Enhancement: EDA often involves collaboration with domain experts who can provide valuable insights. This fusion of data analysis and domain knowledge can lead to a deeper understanding of the data and more meaningful results.
Pattern Recognition: Through EDA, you can unveil hidden patterns and relationships that may not be apparent at first glance. These insights can be pivotal for businesses, researchers, and decision-makers.
Hypothesis Formulation: EDA can trigger the formulation of hypotheses. For instance, by observing a strong correlation between two variables, one can hypothesize that they are causally related and design experiments to test this hypothesis.
Communication: EDA is not only for data scientists and analysts but also for effective communication with stakeholders. Visualizations and summaries generated during EDA can be shared to convey the story the data is telling.
The Power of EDA
Ensuring Data Quality: By identifying and addressing data quality issues, EDA ensures that the subsequent analysis and modeling are based on reliable and accurate data.
Mitigating Risks: EDA helps in identifying potential risks and uncertainties associated with the data, enabling proactive measures to be taken to mitigate these risks.
Enabling Better Decision-Making: Through a deeper understanding of the data, EDA enables stakeholders to make informed decisions, minimizing the likelihood of making decisions based on incomplete or misleading information.
Enhancing Predictive Accuracy: EDA aids in improving the accuracy of predictive models by selecting relevant features, handling outliers, and identifying patterns that contribute significantly to the predictive power of the model.
Optimizing Business Operations: By revealing insights into customer behavior, market trends, and operational inefficiencies, EDA helps businesses optimize their strategies and operations for improved performance and competitiveness.
Driving Innovation and Discovery: EDA can uncover unexpected patterns or relationships in the data, leading to new insights, innovations, and discoveries that may have remained hidden without thorough exploration.
Reducing Costs and Wastage: By identifying areas of inefficiency or waste within the data, EDA can help in reducing unnecessary costs and optimizing resource allocation.
Enhancing Communication: EDA facilitates effective communication between data analysts and stakeholders by providing visually appealing representations of complex data, making it easier for non-technical audiences to grasp the key findings.
Key Tips for Effective EDA
-
Stay Organized: Maintain a systematic approach to EDA by keeping detailed notes, recording the steps taken, and organizing the findings in a structured manner to ensure easy reference and reproducibility.
-
Iterative Exploration: Approach EDA as an iterative process, revisiting and refining the analysis as new insights are uncovered or as new data becomes available, ensuring a comprehensive understanding of the dataset.
-
Balance Automation and Manual Inspection: While leveraging automation tools for routine tasks, also allocate time for manual inspection and verification to ensure a thorough understanding of the data nuances that automated tools might overlook.
-
Collaborate with Domain Experts: Engage with domain experts or stakeholders to gain a deeper understanding of the context and domain-specific insights, which can enrich the analysis and provide a more holistic perspective.
-
Utilize Multiple Perspectives: Employ various visualization techniques and statistical methods to examine the data from different perspectives, enabling a comprehensive exploration of the data distribution, patterns, and relationships.
-
Document Assumptions and Limitations: Document the assumptions made during the analysis and be transparent about the limitations of the data, acknowledging any potential biases or constraints that might impact the findings.
-
Ensure Data Privacy and Security: Adhere to data privacy and security protocols throughout the EDA process, especially when dealing with sensitive or confidential information, to maintain the integrity and confidentiality of the data.
-
Communicate Findings Effectively: Translate complex findings into clear, concise, and compelling narratives that resonate with the intended audience, utilizing intuitive visualizations and straightforward visuals to convey the key insights derived from the EDA.
Exploratory Data Analysis is like a compass that guides you through uncharted data territory. It helps you navigate the complexities of raw data, uncover hidden insights, and make informed decisions. EDA turns data into actionable knowledge, providing a deep understanding of the dataset, a clear roadmap for data preprocessing, and a set of visualizations to communicate findings. In today's data-driven world, EDA is the foundation for all subsequent analysis tasks, from improving machine learning models to making data-informed business decisions. It transforms data into meaningful insights, making it an indispensable tool for any data analyst. So, don't forget to use the magic of EDA on your next data analysis adventure and witness the enchanting transformation of raw data into actionable insights.