AI and Explainability
Understand the importance, challenges, and methods for making AI decisions more transparent and understandable.
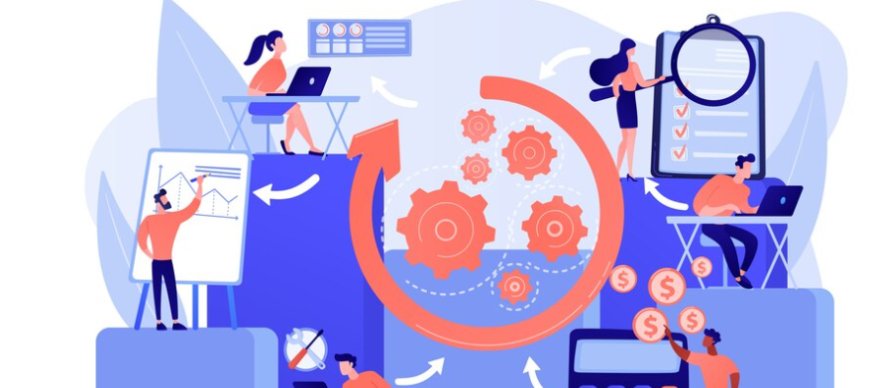
Artificial intelligence (AI) has rapidly transformed various industries, revolutionizing the way we approach complex tasks and processes. From predictive algorithms to autonomous decision-making systems, AI has the potential to significantly enhance efficiency and innovation. However, as AI systems become increasingly sophisticated, the challenge of ensuring their transparency and explainability has emerged as a critical concern. This blog will explore the significance of AI explainability, its current implications across different sectors, the challenges associated with achieving explainable AI, and the potential solutions to strike a balance between AI advancement and comprehensibility.
As AI applications continue to proliferate in domains such as healthcare, finance, and law, the need for transparent and interpretable AI models has become paramount. In healthcare, for instance, the deployment of AI-powered diagnostic tools and treatment recommendations necessitates clear explanations of how AI arrives at its conclusions. Similarly, in the financial sector, the use of AI-driven algorithms for risk assessment and investment decisions demands comprehensible justifications for the outcomes generated. The absence of explainability in these AI systems not only hinders the trust of end-users and stakeholders but also raises ethical and legal concerns, particularly in cases where critical decisions impact human lives and livelihoods.
Let's break down the challenges associated with achieving AI explainability into distinct points:

Natural Complexity of Deep Learning Models:
-
Deep learning and neural network models often function as black boxes, making it difficult for humans to comprehend the underlying mechanisms driving their decisions.
-
The intricate layers of computations and transformations within these models obscure the interpretability of the decision-making process, posing a significant challenge for researchers and developers striving to achieve transparency.
Trade-off Between Model Accuracy and Interpretability:
-
As AI models become more complex to improve accuracy and predictive performance, they tend to sacrifice interpretability.
-
The pursuit of higher accuracy often comes at the cost of reduced explainability, creating a dilemma for practitioners who must balance the need for precise predictions with the requirement for comprehensible and transparent decision-making processes.
Absence of Standardized Frameworks for Assessing Explainability:
-
The lack of universally accepted frameworks and methodologies for evaluating the explainability of AI systems hinders the establishment of standardized guidelines.
-
Without clear and consistent criteria for assessing the transparency and comprehensibility of AI models, developers and stakeholders face difficulties in ensuring that AI systems meet ethical and regulatory standards.
Ethical and Legal Implications:
-
The opacity of AI decision-making processes raises ethical concerns, especially in critical domains such as healthcare, finance, and criminal justice, where AI-driven recommendations and decisions can significantly impact individuals' lives and well-being.
-
The potential for biased or unjust outcomes due to the opacity of AI models necessitates the development of robust ethical and legal frameworks to mitigate risks and safeguard against discriminatory or unfair practices.
Interpretability Techniques and Tools:
-
While various techniques exist for interpreting and explaining AI model outputs, their efficacy and applicability may vary depending on the complexity of the model and the specific use case.
-
The selection and implementation of appropriate interpretability tools require specialized expertise and resources, posing a challenge for organizations seeking to enhance the transparency and accountability of their AI systems.
User Trust and Acceptance:
-
The lack of transparency and interpretability in AI systems can undermine user trust and acceptance, particularly in applications where users rely on AI-driven recommendations or decisions.
-
Building user confidence in AI technologies requires clear and accessible explanations of how AI arrives at its conclusions, enabling users to understand and trust the reasoning behind AI-generated outputs.
How can we address the challenges associated with AI explainability without compromising the potential advancements and capabilities of AI systems?
Successfully addressing the challenges of AI explainability involves a holistic approach that focuses on integrating interpretable AI models, establishing standardized frameworks for explainability, and enforcing regulatory policies that promote transparency and accountability. This comprehensive strategy aims to ensure that AI systems are not only accurate but also understandable and ethically responsible, thereby building trust and confidence in their applications across different industries. By combining these essential elements, we can create a more transparent and responsible AI landscape, encouraging innovation while upholding ethical and legal standards.
Utilization of Interpretable AI Models:
-
Inherently interpretable AI models, like decision trees and linear models, offer transparency and comprehensibility by design. Unlike complex deep learning models, they provide clear insights into how they arrive at conclusions.
-
Prioritizing these models empowers businesses and researchers to balance accuracy and explainability, ensuring that AI systems can be understood by end-users and stakeholders. This transparency fosters trust and confidence in the technology.
Integration of Model-Agnostic Interpretability Techniques:
-
Model-agnostic techniques such as feature importance analysis and sensitivity analysis are valuable tools for understanding AI decision-making processes. These techniques can be applied to various models, enhancing their transparency.
-
Feature importance analysis helps users gain insights into which factors influence AI predictions, while sensitivity analysis allows for a deeper understanding of how changes in input data affect model outcomes. This empowers users to trust and interpret AI decisions.
Establishment of Standardized Explainability Frameworks and Guidelines:
-
The development of clear and standardized frameworks and guidelines for evaluating AI explainability is essential. It ensures that AI models adhere to ethical and legal standards consistently.
-
Regulators and industry bodies play a crucial role in defining benchmarks and criteria. This approach minimizes the risks associated with biased or untrustworthy AI outputs, creating a more transparent and accountable AI landscape.
Implementation of Explainability Audits and Certifications:
-
Explainability audits and certifications can encourage developers to prioritize transparency and accountability throughout the AI development process. These audits can be conducted by independent organizations or regulatory bodies.
-
When developers seek and obtain certification for their AI systems, it signals their commitment to responsible AI innovation. This fosters a culture where explainability is a key consideration.
Integration of Regulatory Policies and Ethical Guidelines:
-
Government involvement is crucial in enhancing the overall transparency and trustworthiness of AI applications. Mandating the disclosure of AI decision-making processes and the documentation of model training data and algorithms ensures accountability and transparency.
-
These policies protect the interests of consumers, ensuring that AI is used ethically and responsibly. It also provides legal recourse in cases of AI-related issues.
Promotion of Interdisciplinary Collaboration:
-
Collaboration between AI developers, ethicists, and legal experts is key to comprehensive governance frameworks. These frameworks address not only the technical but also the ethical, social, and legal implications of AI explainability.
-
By bringing multiple perspectives together, responsible AI innovation is promoted, and potential ethical and legal issues are proactively addressed. This collaborative effort ensures that AI benefits society while mitigating potential risks.
-
Incorporating these solutions into AI development and deployment practices can create a more transparent, accountable, and trustworthy AI ecosystem. This, in turn, fosters confidence among users, addresses ethical concerns, and ensures that AI is harnessed for the betterment of society while mitigating potential risks and challenges.
It is crucial to prioritize AI explainability, transparency, and accountability alongside the pursuit of AI advancement. Despite ongoing challenges like model complexity and the lack of standardized frameworks, we can build trust and comprehension in AI systems by incorporating interpretable AI models, establishing clear regulatory policies, and implementing standardized explainability guidelines. By finding the right balance between technological innovation and ethical concerns, we can harness the transformative potential of AI while ensuring the well-being of society and promoting responsible and transparent AI development and deployment.