Understanding the Key Stages of AI Development
Explore the evolution of AI with insights into its key development stages, from inception to future possibilities. Learn about AI history, growth, and trends.
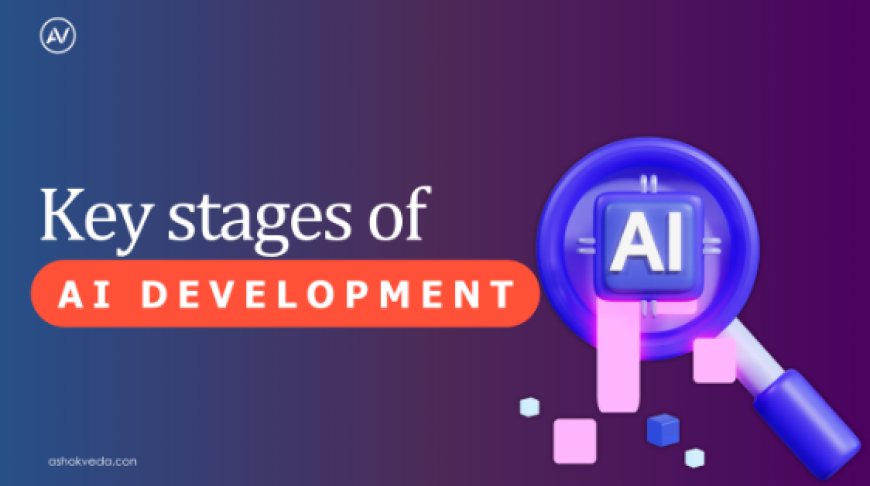
The concept of artificial intelligence (AI) implies a machine's capacity to imitate thoughtful human behavior. To understand how these technologies develop and become essential components of our everyday lives, it is imperative to understand the Key Stages of AI Development. Large volumes of data are collected in the first phase of data collection to train AI systems. Machine learning, which uses computers to learn from data patterns and make judgments with little to no human input, comes next. Understanding the Important Stages of Artificial Intelligence Development includes the development of neural networks, which replicate the architecture and operations of the human brain, empowering machines to identify patterns and resolve intricate issues. Natural language processing is an essential phase that enables AIs to understand and produce human language, improving communication. AI has a significant impact on many industries, including healthcare and the automotive. Additionally, it is essential for improving production efficiency and advancing research and development. AI is becoming more integrated in supporting and changing numerous aspects of modern life as it grows.
Stage 1: Rule-Based Systems
Rule-based AI operates on a set of predefined rules to make decisions. These rules, formulated as "if-then" statements, dictate how the system should respond to various inputs. It is developed in the 1970s and 1980s, rule-based systems were among the first forms of AI. These systems were primarily used in expert systems and early artificial intelligence applications to simulate human expert knowledge in specific domains, such as medicine and engineering.
Rule-Based Systems' Limitations in Managing Complicated Tasks:
-
They find it difficult to complete tasks that call for context awareness or complexity beyond the parameters they have set.
-
Rule-based systems limit flexibility since they require human rule set modifications to learn from fresh data or experiences.
-
When making decisions using unclear or insufficient data, their usefulness is limited.
Stage 2: Machine Learning
Machine Learning (ML) is a subset of AI that enables systems to learn and improve from experience without being explicitly programmed. It focuses on the development of algorithms that can interpret data, learn from it, and make decisions based on the learned knowledge.
Key Developments that Shifted AI from Rule-Based Systems to ML Models:
-
The development of neural networks offered a foundation for creating algorithms capable of simulating the levels of information processing in the human brain.
-
Late 20th-century developments in computing power and data storage made it possible to train increasingly complicated models on bigger datasets.
-
The creation of backpropagation algorithms, allowed neural networks to gradually increase their accuracy and modify their parameters.
Various ML Techniques:
Supervised learning: Supervised learning is a technique that teaches a model to predict the output from the input data by using a labeled dataset where the right output is known.
Unsupervised learning: Unsupervised learning is the process of teaching a model with data that is neither classified nor labeled. The model is then left to operate on the data on its own, looking for natural structures or hidden patterns.
Reinforcement learning: Reinforcement learning is an approach that teaches an agent how to respond in a given environment by having it do certain things and then observing the outcomes, which are then interpreted as rewards or penalties and help the agent choose the best course of action.
Stage 3: Deep Learning and Neural Networks
Deep learning is a specialized area within machine learning that uses neural networks to mimic human-like decision-making. These networks consist of layers of nodes that process data, learning from vast amounts of information to perform complex tasks.
Key Advances from Deep Learning:
-
Image Recognition: Uses networks to accurately identify and classify images across various applications, such as facial recognition and social media.
-
Speech Recognition: Enhances machine understanding of human speech, supporting developments like voice-activated assistants and real-time translation.
Stage 4: Cognitive Computing
Cognitive computing aims to imitate human thought and intelligence. It creates systems with natural language interpretation, reasoning, learning, and human-like interaction capabilities by combining artificial intelligence and signal processing. The goal of cognitive computing is to support decision-making through the combination of data from several sources while taking uncertainty and context into consideration. Numerous industries employ this technology, including healthcare for diagnosis and treatment suggestions, financial services for fraud detection and risk management, and customer service for chatbots.
Differences between cognitive computing and other AI stages
Aspect |
Cognitive Computing |
Other AI Stages (e.g., Machine Learning, Deep Learning) |
Objective |
To simulate human thought processes in complex situations. |
To automate tasks that typically require human intelligence, focusing on specific tasks. |
Learning and Adaptation |
Mimics of human reasoning learn from interaction and can adapt based on new information. |
Typically uses algorithms to learn from data without human-like reasoning or adaptation. |
Interaction |
Uses natural language processing to interact in human-like ways. |
May not be designed for natural human-like interaction. |
Application |
Used in decision-making support with reasoning capabilities. |
Applied in pattern recognition, data analysis, and repetitive tasks without intrinsic reasoning. |
Data Processing |
Handles unstructured data and interprets context and ambiguity. |
Often relies on structured data and specific, clear inputs without ambiguity. |
Stage 5: Autonomous AI Systems
AI systems that are capable of making decisions independently. These technologies, which range from completely automated financial trading platforms to autonomous cars, are made to function without human supervision. Since autonomous AI systems must make decisions that comply with social norms and ethical standards, ethical considerations are crucial. This presents serious regulatory issues as legislators attempt to create structures that guarantee responsibility and safety. Autonomous AI has enormous promise in the future. It is anticipated that these systems will become more adaptive and capable of self-learning, allowing them to change and adapt to their surroundings and needs. This development has the potential to improve efficacy and efficiency in several areas, but it must be carefully managed to reduce any concerns related to autonomy.
The Future of AI
Advanced systems that are highly effective and efficient at dealing with challenging problems are probably going to be created as a result of the merging of different AI stages. The confluence of rule-based systems, machine learning, and deep learning will make it possible to create AI systems with improved learning, reasoning, and decision-making skills. Through this integration, AI and humans will be able to communicate more naturally as systems with real-time problem-solving, context awareness, and situational awareness evolve. As these linked systems acquire autonomy, ethical AI development will become more and more crucial, requiring strong frameworks to guarantee that they function within social standards. Additionally, the combination of AI stages is expected to spur advances in a variety of industries, including healthcare, where improved patient diagnosis and treatment will be possible, and transportation, where smarter, safer self-driving cars. This complete development of AI will increase its potential applications while also improving its efficacy in already-existing fields.
In conclusion, the stages of AI development trace the evolution from basic, rule-based systems to advanced autonomous technologies. Each stage has introduced new capabilities: rule-based systems applied simple, predefined rules; machine learning enabled AI to learn from data; deep learning enhanced pattern recognition; cognitive computing allowed systems to mimic human thought processes; and autonomous AI systems are now making decisions independently. These stages illustrate significant advancements in AI's ability to process information and interact with the world. As AI continues to evolve, it’s crucial to consider ethical issues and develop appropriate regulations to ensure these technologies are used responsibly and safely.