Power of Big Data with Machine Learning
Unlock the immense potential of big data through the power of machine learning
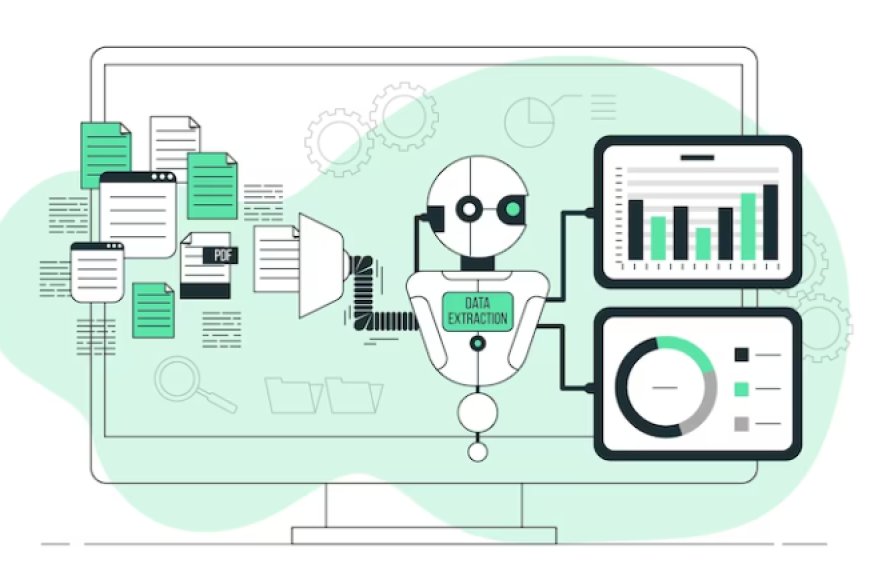
In today's digital age, the exponential growth of data has presented both challenges and opportunities. Big data, characterized by its volume, velocity, and variety, has become a valuable resource for organizations across various industries. But how can we extract meaningful insights from this vast sea of information? Enter machine learning, a powerful approach that enables us to unlock the potential of big data. In this blog, we'll explore the intersection of big data and machine learning and delve into how they complement each other to drive innovation and decision-making.
The Era of Big Data
In today's digital landscape, we are living in the era of big data. The sheer volume, velocity, and variety of data being generated have transformed the way we understand and interact with information. Every click, transaction, social media post, sensor reading, and communication contributes to this massive data ecosystem. Organizations across industries have access to unprecedented amounts of data, providing an opportunity to gain deep insights and drive informed decision-making.
The rise of big data has been fueled by advancements in technology, including cloud computing, the Internet of Things (IoT), and the proliferation of digital devices. These developments have made it possible to collect, store, and process vast amounts of data efficiently and cost-effectively. Additionally, the increasing digitization of various aspects of our lives has resulted in an exponential growth in data creation.
Big data encompasses structured, semi-structured, and unstructured data, coming from diverse sources such as social media, weblogs, sensors, images, videos, and more. This wealth of data holds immense value, as it provides organizations with the potential to uncover hidden patterns, trends, and insights that can drive innovation, optimize operations, and enhance customer experiences.
However, big data also presents challenges. The sheer volume and complexity of data make it difficult to manage, process, and analyze using traditional methods. Organizations need to invest in scalable storage and computing infrastructure, as well as advanced analytical tools and techniques to effectively harness the power of big data.
Moreover, privacy and security concerns arise as more personal and sensitive information is collected and stored. Safeguarding data privacy and implementing robust security measures are of utmost importance to maintain trust and protect individuals' rights.
In this era of big data, organizations that can effectively harness and analyze data stand to gain a competitive advantage. By leveraging advanced analytics, machine learning, and artificial intelligence techniques, they can unlock valuable insights, make data-driven decisions, and stay ahead in the rapidly evolving digital landscape.
As big data continues to grow exponentially, it will shape various aspects of our lives, from personalized experiences and improved healthcare to smart cities and data-driven policymaking. Embracing the era of big data opens up endless possibilities for innovation, discovery, and societal transformation.
Machine Learning: Transforming Big Data into Insights
In the era of big data, organizations have access to vast volumes of data from diverse sources. However, the true value lies in transforming this data into actionable insights. By leveraging advanced analytics, machine learning, and artificial intelligence techniques, organizations can uncover patterns, trends, and relationships within big data that drive informed decision-making. This process involves scalable storage and computing infrastructure, robust data governance practices, and the ability to extract meaningful insights from structured and unstructured data. Transforming big data into insights enables organizations to optimize operations, enhance customer experiences, and gain a competitive edge in today's data-driven landscape.
Applications of Machine Learning in Big Data
The combination of machine learning and big data has opened up a plethora of possibilities across various industries. By leveraging machine learning algorithms and techniques, organizations can extract valuable insights from vast amounts of data and drive innovation. Here are some key applications of machine learning in the realm of big data:
1. Predictive Analytics:
Machine learning excels in predictive analytics by leveraging historical and real-time data to forecast future trends and events. Organizations can utilize machine learning algorithms to analyze big data and make accurate predictions about customer behavior, market demand, financial trends, and more. This enables businesses to optimize their operations, identify potential risks, and make informed strategic decisions.
2. Fraud Detection:
In industries such as finance, insurance, and e-commerce, detecting fraudulent activities is crucial. Machine learning algorithms can analyze large volumes of transactional data, identifying patterns and anomalies that indicate potential fraud. By leveraging advanced fraud detection models, organizations can mitigate risks, prevent financial losses, and protect their customers from fraudulent activities.
3. Personalized Recommendations:
Machine learning algorithms are highly effective in understanding user preferences and providing personalized recommendations. In e-commerce, streaming services, and online platforms, machine learning models analyze user behavior, purchase history, and social interactions to offer tailored product recommendations, content suggestions, and personalized experiences. This enhances user satisfaction, engagement, and drives revenue growth.
4. Natural Language Processing (NLP):
Big data often includes a vast amount of unstructured textual data, such as social media posts, customer reviews, and support tickets. Natural Language Processing (NLP) techniques, a subfield of machine learning, enable organizations to extract valuable insights from this unstructured data. NLP algorithms can perform sentiment analysis, topic modeling, text classification, language translation, and chatbot interactions, facilitating efficient data analysis and decision-making.
5. Customer Churn Prediction:
Reducing customer churn is a critical objective for many businesses. Machine learning models can analyze customer data, including past interactions, behavior patterns, and usage metrics, to predict the likelihood of customers churning. By identifying at-risk customers in advance, organizations can take proactive measures to retain them, such as personalized offers, targeted marketing campaigns, or improved customer service.
6. Image and Video Analysis:
With the proliferation of visual content, machine learning plays a vital role in analyzing images and videos at scale. Machine learning models can be trained to recognize objects, faces, gestures, and scenes within images and videos. This enables applications such as automated image tagging, object detection, facial recognition, and video content analysis for various purposes, including security surveillance, autonomous vehicles, and medical imaging.
7. Healthcare Analytics:
Machine learning in healthcare analytics has the potential to revolutionize patient care, disease diagnosis, and drug discovery. By analyzing large volumes of medical data, including electronic health records, medical images, and genomics data, machine learning models can aid in early disease detection, personalized treatment recommendations, and drug development. This can improve patient outcomes, optimize resource allocation, and accelerate medical research.
Challenges and Considerations
While the combination of big data and machine learning offers immense potential, it also poses challenges. Some of the key considerations include:
1. Data Quality: Ensuring data accuracy, completeness, and reliability is crucial for reliable machine learning results. Garbage in, garbage out. It is vital to establish data governance practices and implement data quality checks to ensure the integrity of the data.
2. Scalability and Infrastructure: Processing and analyzing big data require robust computational infrastructure capable of handling the volume, velocity, and variety of data. Distributed computing frameworks, cloud platforms, and specialized hardware accelerators can help address scalability challenges.
3. Privacy and Security: With the abundance of personal and sensitive information in big data, privacy and security concerns arise. Organizations must prioritize data protection, implement strong security measures, and adhere to relevant regulations and compliance standards.
The convergence of big data and machine learning has revolutionized the way we analyze and utilize data to drive insights and innovation. By leveraging machine learning algorithms and techniques, organizations can extract valuable knowledge from vast amounts of data, enabling them to make data-driven decisions, gain a competitive edge, and deliver personalized experiences to their customers. As we continue to generate more data, the synergy between big data and machine learning will play a pivotal role in shaping the future of various industries and driving transformative advancements in technology and society.