Security Problems of Artificial Intelligence
Learn the challenges of artificial intelligence security, including data breaches, algorithm vulnerabilities, and ethical concerns.
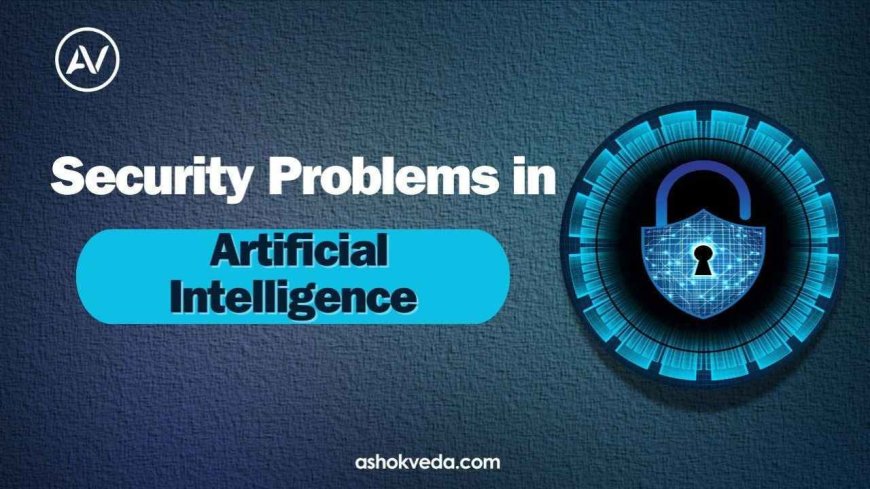
Hello! We will look at the security issues around Artificial Intelligence. As AI becomes more integrated into our lives, from smartphones to healthcare, we need to understand the problems it faces, particularly in terms of security.These security problems of artificial intelligence Can have serious effects if not addressed properly. Imagine the effects of someone hacking into an AI system that operates your vehicle or manages your personal information. We'll look at what these security issues are, why they matter, and how we can work towards solving them. By understanding these risks, we can better protect ourselves and the systems We depend on AI security and learn how to keep our digital future safe.
Understanding Security Risks in AI
Let's identify security risks in AI. AI is actually based on data. It learns from the facts it is given, makes decisions, and develops over time. But what if that data gets into the wrong hands? Worse, what if someone manages to change the data? These are some of the core security issues with Artificial Intelligence.
Data thefts present an important danger. AI systems frequently deal with large amounts of sensitive data, which includes personal details to financial records. If hackers gain access to this information, they may create serious damage. Another issue is data poisoning, in which criminals actively feed incorrect information into AI systems to manipulate results or cause failures.
Also, AI systems are becoming more complicated, making them harder to safeguard. As they interact with different data sources and integrate into several platforms, the attack surface grows. This means there are more potential access points for criminals to use.
Types of Security Threats in AI
AI systems face a variety of security threats, each with its own potential impact.
Data incidents: As previously stated, AI systems manage a large amount of data. Improper access to sensitive data might result in breaches of privacy and financial loss. In many cases, sensitive information such as medical records, financial information, and personal identification are at danger, making data breaches more harmful.
Negative Attacks: These are sophisticated attacks in which adversaries use false data to fool AI models into making inaccurate predictions. For example, changing a few pixels in an image can cause an AI system to misclassify it. This type of attack can have major effects in applications such as automated driving, where poor decisions can result in accidents.
Model Theft: Creating AI models requires outstanding resources. If these models are taken, they can be utilized criminally in addition to causing loss of intellectual property. Competitors or bad actors can reverse-engineer these models to devise counter-strategies or exploit the system.
AI Strategies: These involve exploiting flaws in AI algorithms. For example, an attacker may discover a flaw in the way an AI system analyzes data and exploit it to get unwanted access. Utilizing these flaws can result in control over vital systems or access to sensitive information.
Bias & Discrimination: Although biased AI systems do not provide a direct security danger, they can treat individuals or groups unfairly, resulting in social and ethical issues. Bias in AI might result in unbalanced training data, leading to decisions that discriminate against certain populations.
Case Research on Hacking in AI
Healthcare Data Theft: In 2020, a major healthcare provider's AI system was hacked, exposing sensitive patient data. This attack exposed the weaknesses of AI systems that handle sensitive health data. The attackers got access by targeting an opening in the data processing system, highlighting the need to safeguard all points of data entry.
Automatic Vehicles: In some cases, adversarial attacks caused self-driving cars to misread road signs. In one case, researchers were able to trick an AI system into believing a stop sign was a yield sign by applying stickers to it. This manipulation has the potential to cause accidents, highlighting the importance of strong security measures in AI systems used in transportation.
Financial Services: Artificial intelligence algorithms in banking have been targeted for fraud. Hackers modified transaction data, leading the AI system to approve fake transactions. This incident demonstrates how weaknesses in financial AI systems might be abused to generate huge monetary gains.
Facial Recognition: Criminal attacks on face recognition systems have demonstrated that by introducing minor, unnoticeable alterations to facial images, attackers can cause the system to misidentify people. This represents an important threat to security systems that rely on facial recognition for access control.
Social Media Manipulation: AI-powered social media platforms have been used to disseminate misinformation and sway public opinion. By generating and promoting fake content, attackers might influence public opinion and instigate societal instability.
These incidents show that artificial intelligence security issues are not purely theoretical, but also have real-world implications. They influence a variety of industries and can have far-reaching implications if not addressed effectively.
Solving AI Security Issues
Now that we've identified potential risks, what can we do about them? Here are some solutions to reduce the security issues in AI:
1.Robust Data Security: Using robust encryption and access restrictions to keep data safe from unauthorized access. Ensuring that data is encrypted at rest and in transit can dramatically reduce the chance of an attack on data.
2.Regular Audits: Conducting regular security audits on AI systems to find and address issues. Regular audits can help identify system flaws and resolve them before they are abused.
3.Adversarial Training: Adversarial training is using adversarial instances to improve AI models' resilience to attacks. Exposing AI systems to potential assault situations allows them to learn to recognize and reject such efforts.
4.Transparency and Accountability: Ensure that AI systems operate transparently and that AI judgments are accountable. Transparent systems make it easier to discover and correct problems, whereas accountability ensures that there is a procedure for dealing with any harm caused by AI judgments.
5.Ethical AI Practices: Creating AI with a focus on justice, accountability, and transparency in order to reduce flaws and assure ethical application. Implementing rules and standards for social AI development may help in the creation of systems that are both effective and fair.
6.Multi-layered Security: To protect AI systems, a multi-layered security approach is used, which includes antivirus programs, attack detection systems, and regular updates. This structured strategy ensures that if one security measure fails, the others can still provide protection.
7.User Education: Educating users on the potential risks and best practices for using AI systems safely. Users' awareness can help them recognize and report questionable actions, contributing to overall security.
The Role of Policy and Government
Addressing artificial intelligence security issues requires more than just technology; politics and law must also be considered. Governments and legal things play important parts in developing AI security standards and guidelines. Here's how regulations and laws can help:
Existing Policies and Regulations
There are already certain restrictions in place to protect data and keep AI systems secure. For example, in Europe, the General Data Protection Regulation (GDPR) establishes strict data privacy and security requirements. Similar policies are being drafted and implemented around the world.
Government Oversight
Governments may help by providing monitoring and ensuring that enterprises and organizations conform to AI security best practices. This includes creating rules for the secure development and operation of AI systems, as well as conducting regular audits and assessments.
International Cooperation
AI is a global technology, and solving its security challenges requires worldwide collaboration. Countries can collaborate to share knowledge, create common standards, and address cross-border security concerns. Collaborative efforts can result in more efficient solutions and a safer AI ecosystem.
Security problems of artificial intelligence are an important worry as these technologies become more integrated in our daily lives. The dangers are broad and difficult to manage, ranging from adversarial attacks and data privacy concerns to model inversion attacks. We may limit these risks by using strong development techniques, conducting regular audits and testing, and employing strong data encryption mechanisms. Policy and regulation, as well as government monitoring and international cooperation, are critical for maintaining the security of AI systems.
By taking action on these security issues, we may optimize the benefits of artificial intelligence while protecting our data and systems. Let's be informed, aware, and work to create a secure future with AI.