Guide to Quantum Machine Learning
Explore quantum computing, machine learning, algorithms, data, integration, models, research, applications, advances, and technologies.
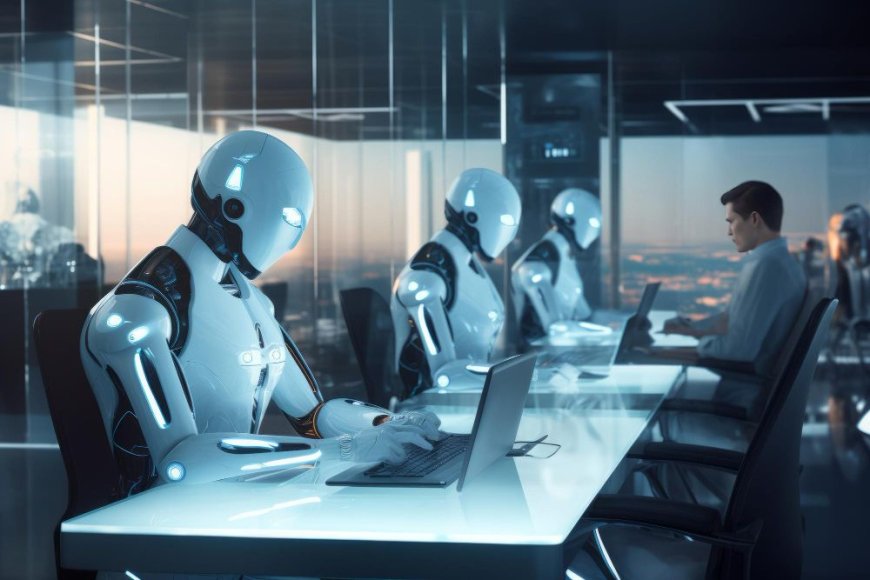
As the fields of artificial intelligence (AI) and quantum computing continue to advance, the intersection of these two domains has given rise to the emergence of quantum machine learning (QML). Quantum machine learning represents a revolutionary approach to processing and analyzing complex datasets, leveraging the unique properties of quantum mechanics to enhance the capabilities of traditional machine learning algorithms. This convergence of AI and quantum computing has the potential to unlock new frontiers in data analysis, pattern recognition, and problem-solving, paving the way for unprecedented advancements in various industries, including healthcare, finance, and materials science.
Challenges in Integrating Quantum Computing with Machine Learning
-
Complexity of Quantum Algorithms: Developing effective quantum algorithms poses a significant challenge due to their intricate nature, requiring a deep understanding of quantum principles and mathematics. Designing algorithms that can efficiently process complex data sets and perform advanced computations demands specialized expertise and a comprehensive grasp of quantum mechanics.
-
Need for Specialized Hardware: Quantum machine learning relies on specialized quantum hardware that can manipulate and process quantum information effectively. The development and maintenance of such hardware demand substantial investments in research and infrastructure, limiting the accessibility of quantum computing resources for organizations and researchers.
-
Requirement for Skilled Quantum Computing Experts: The successful implementation of quantum machine learning requires a skilled workforce proficient in both quantum computing and machine learning. The scarcity of professionals with expertise in both domains poses a challenge, as the training and education required to bridge this gap are extensive and time-consuming.
-
Sensitivity of Quantum Systems to Noise and Errors: Quantum systems are highly sensitive to external noise and errors, leading to decoherence and loss of computational accuracy. Ensuring the reliability and stability of quantum machine learning models necessitates the implementation of robust error correction techniques and fault-tolerant quantum hardware, adding complexity and cost to the development process.
-
High Cost of Quantum Computing Infrastructure: The significant financial investment associated with establishing and maintaining quantum computing infrastructure acts as a deterrent for many organizations. The high cost of hardware, research, and development in the field of quantum computing limits the widespread adoption of quantum machine learning, particularly for smaller enterprises and research institutions with limited resources.
-
Limited Accessibility of Quantum Hardware: The accessibility of quantum hardware remains limited, with only a handful of companies and research facilities possessing the necessary resources and expertise. The lack of widespread availability and accessibility of quantum computing hardware restricts the opportunities for experimentation and research, hindering the advancement and practical application of quantum machine learning technologies.
How can quantum machine learning revolutionize the AI and data analysis environment, driving innovation and breakthroughs in various fields? What advancements in quantum algorithms and hardware development are necessary to overcome the limitations of quantum machine learning and make it practical? How can integrating quantum machine learning lead to a more efficient and advanced approach to solving complex real-world problems and promote the accessibility of quantum computing technologies?
Enhanced Data Analysis and Pattern Recognition:
Quantum machine learning can revolutionize data analysis by processing and analyzing vast datasets with unprecedented speed and efficiency. Leveraging the principles of quantum superposition and entanglement, quantum machine learning algorithms can identify complex patterns and correlations within data, enabling more accurate predictions and insights for decision-making processes
-
Quantum computers can perform data analysis tasks, such as clustering and classification, exponentially faster than classical computers. This speed can significantly impact industries like healthcare and finance by enabling quicker insights into patient diagnoses and investment strategies.
-
Quantum algorithms, such as the Quantum Support Vector Machine (QSVM), can recognize subtle patterns in large datasets, which is particularly beneficial in areas like materials science and drug discovery.
Optimized Optimization and Simulation:
By harnessing the computational power of quantum computing, quantum machine learning can expedite optimization and simulation processes for complex systems and models. Quantum algorithms can efficiently solve optimization problems, simulate molecular interactions, and optimize resource allocation, leading to faster and more precise solutions for complex real-world problems.
-
Quantum computers excel in solving optimization problems by leveraging quantum annealing. This can have applications in logistics, supply chain management, and resource allocation, where finding the most efficient solutions is paramount.
-
Quantum simulations of molecular interactions enable the accurate modeling of complex chemical reactions, which is valuable for drug discovery and material design.
Secure and Private AI Solutions:
Quantum machine learning offers the potential to develop secure and private AI solutions through the implementation of quantum encryption and secure computation techniques. Quantum cryptography can enhance data security and privacy, ensuring that sensitive information remains protected from potential cyber threats and unauthorized access, thereby fostering a more secure and reliable digital ecosystem.
-
Quantum key distribution (QKD) allows for unbreakable encryption, making it highly attractive for secure communication, especially in industries dealing with sensitive data, like healthcare and finance.
-
Quantum secure multi-party computation (SMPC) enables privacy-preserving AI solutions where multiple parties can jointly analyze data without revealing their individual inputs. This is crucial in collaborative research and data-sharing scenarios.
Robust Quantum Error Correction and Fault-Tolerance:
Advancements in quantum error correction and fault-tolerant quantum computing are critical to overcoming the challenges associated with noise and errors in quantum systems. By developing robust error correction protocols and fault-tolerant quantum hardware, researchers can enhance the reliability and stability of quantum machine learning models, ensuring the accuracy and consistency of computational results for a wide range of applications.
-
Quantum error correction codes, such as surface codes, help mitigate the effects of noise and errors, improving the fidelity of quantum computations. This is vital for ensuring the practicality of quantum machine learning.
-
Development of fault-tolerant quantum hardware involves creating qubits that are less susceptible to errors, which is essential for building reliable quantum machines for AI applications.
Accessible Quantum Computing Infrastructure:
The development of accessible and user-friendly quantum computing infrastructure is essential to democratizing quantum machine learning technologies. By creating cloud-based quantum computing platforms and providing educational resources for aspiring quantum computing practitioners, the accessibility and adoption of quantum machine learning can be expanded, fostering a more inclusive and collaborative quantum computing community.
-
Cloud-based quantum computing platforms, like IBM Quantum Experience and Microsoft Azure Quantum, enable users to access quantum hardware and conduct quantum experiments without the need for a local quantum computer.
-
Educational programs and quantum software development kits (SDKs) are critical in lowering the entry barriers for individuals and organizations interested in quantum machine learning. These resources facilitate skill development and knowledge sharing.
Interdisciplinary Research and Collaboration:
Promoting interdisciplinary research and collaboration between quantum computing experts and machine learning practitioners is crucial to fostering innovation and driving advancements in quantum machine learning. By fostering a collaborative environment for knowledge sharing and skill development, researchers can leverage diverse perspectives and expertise to address complex challenges and accelerate the practical implementation of quantum machine learning in various industries and research domains.
-
Collaborative research between quantum physicists and machine learning researchers has led to the development of quantum machine learning algorithms specifically tailored to address real-world problems, such as optimizing energy consumption in data centers or predicting material properties.
-
Multidisciplinary teams bring together experts from quantum physics, computer science, and various application domains to tackle complex challenges, ensuring that quantum machine learning solutions are practical, efficient, and relevant to specific industries.
In summary, the convergence of AI and quantum computing through quantum machine learning represents a paradigm shift in the way we approach data analysis, optimization, and secure computation. By harnessing the unique properties of quantum mechanics, quantum machine learning has the potential to unlock new possibilities for innovation and discovery, revolutionizing various industries and driving the development of advanced solutions for complex real-world problems. However, addressing the challenges associated with quantum machine learning, such as quantum error correction, hardware accessibility, and interdisciplinary collaboration, is crucial to realizing the full potential of this transformative technology. Through continued research, development, and collaboration, we can pave the way for a future where quantum machine learning not only bridges the gap between AI and quantum computing but also empowers us to explore the uncharted territories of scientific discovery and technological advancement.